Connect with execs from The New York Times, TIME, Dotdash Meredith and many more
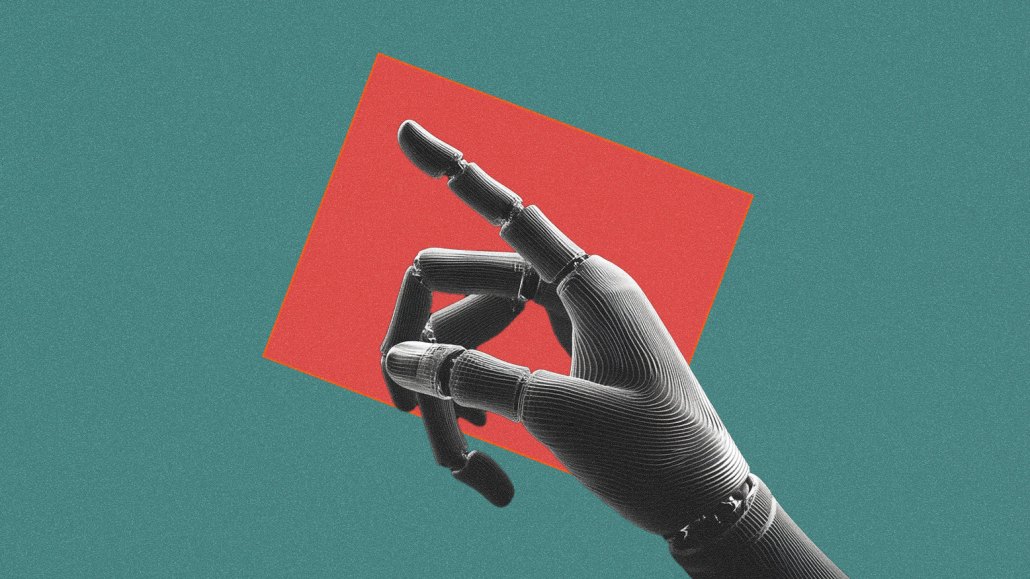
This article is a WTF explainer, in which we break down media and marketing’s most confusing terms. More from the series →
Improving media mix modeling is a top priority for U.S. marketers, according to a 2024 eMarketer study, with 61% claiming they’re working on making MMM “better and/or faster.”
Doubtless, many hope to seize the benefits of AI, with some of the ad industry’s largest names like Amazon, Google and Meta backing open-source projects to help further adoption. Just last month, Google launched a new open-source marketing mix model (MMM) called Meridian, which is designed to help marketers measure performance across media.
Some point to the proponents of open source large language models and hope it’s not a case of adland’s digital oligopoly building a better mousetrap to ensnare marketers’ budgets further — only this time employing the user-privacy narrative as a moat.
It’s worth asking, or even re-asking: What is MMM in the era of online marketing, especially as open-source gains momentum across separate areas like LLMs, AI agents and other applications?
What actually is it, and what are its functions?
Marketers have used MMM for decades. The term entered the industry’s popular lexicon in the late 1980s to early 1990s, but its conceptual roots go back to the 1950s and 1960s as CPGs sought to use econometric modeling to allocate the distribution of media budgets across channels such as OOH, print, radio, and TV.
As marketers began to spend more and more of their advertising budgets online, the ability to apply granular event tracking (i.e., multi-touch attribution, a.k.a. MTA) began to gain popularity from the beginning of the 2010s.
It’s worth noting that while MMM and MTA have the same objectives, they are tactically distinct. For example, the former relies on aggregated data across a period of weeks to months — even years in some circumstances — and factors in several types of marketing interventions.
Meanwhile, MTA employs user-level tracking across a shorter period of time, primarily across digital channels (such as search, social, etc.), to calculate ROAS and optimize budget allocation accordingly. For many, this has led to an over-reliance on last-click attribution modeling, but this is regularly criticized as a two-dimensional approach to allocating value toward specific media channels and is becoming increasingly difficult in the privacy-first era of digital marketing.
Why is ‘MMM’ leading conversations among marketers now?
One of the newest open-source MMMs is Google’s Meridian, which Google previewed last year. It give access to a new MMM data platform that includes info about metrics like impressions, clicks, costs, search query volume, and other metrics, including reach and frequency for video impressions.
Instead of the regression-based models used by traditional MMMs, the Meridian model is based on Bayesian causal inference. This lets marketers combine their own data sources with other campaign data to estimate the incremental impact of campaigns. Google has also created a “Meridian Partner Program” with nearly two dozen initial measurement partners, including Jellyfish, Kinesso, Publicis Media, GroupM, Dentsu, Monks, and Accenture.
While traditional MMMs are often black boxes from third-party vendors, Meridian’s open-source framework promises a way to be more transparent and more privacy-compliant. It also creates new ways for data teams to customize, audit, and refine their models.
Kamal Janardhan, a senior director for Google Ads leading the measurement product team, said Meridian helps customers with more long-range budget allocation. Using Meridian could also help companies measure ads on newer formats like Google’s AI overviews and YouTube TV.
“It’s not just something where you have internal budget allocation decisions, but things like seasonality, pricing, what’s happening in the economy,” Janardhan said. “The aggregated data promise is what allows us to say ‘this will be transparent and protects your data while actually allowing you to process and share it and draw conclusions and insights from it.’”
The rise of open-source MMMs
Open-source models have been around for a while. However, lately there’s been more interest in open-source LLMs, thanks in part to all the hype around open-source LLMs like DeepSeek’s R1, Meta’s Llama models, and LLMs from the French startup Mistral.
By using an open-source framework, the goal is to help advertisers make budget decisions in a more transparent way despite increased signal loss. Google published Meridian MMM on GitHub, which features a repository with codebase, detailed documentation, demos, and configuration files. The page also includes a way to chat with other users, report bugs, and ask for new features.
Meridian isn’t the only model on the market. In 2021, Meta’s open-source, Robyn, debuted to help marketers automate modeling for cross-channel campaigns, including search, CTV, and offline ads. In 2022, Amazon released a self-service MMM for Amazon Ads using Amazon’s first-party data.
So, what do I need to get started?
Gary Danks, general manager, AiM, at Kochava noted that the minimum data requirement to get started is three months — preferably closer to 12 — with inputs including marketing spend breakdown by channel (both online and offline), geography, and corresponding conversion data by platform, etc.
“Next-generation SaaS MMM solutions have faster onboarding times than traditional MMM models, which can take six to 12 months to build,” he said, noting how they can be fully onboarded in approximately 50 days. “While traditional MMM provides only quarterly or bi-annual model refreshes, a SaaS MMM platform updates with daily data to provide always-on insights for weekly model refreshes.”
This sounds great, but are there any catches?
Meridian’s open-source nature gives advertisers ways to adjust model assumptions with their own data, historical incrementality and new inputs like Google search volume. However, some marketing experts wonder if the MMM could still bias Google-owned platforms, creating potential doubts about attribution and model results.
Some point to a pattern of behavior by some of the purveyors of these open-source MMM models. For example, Google bought attribution company Adometry in 2014, with its subsequent changes to the outfit’s measurement program leading to accusations of bias.
Another challenge for the industry is having enough talent for data teams to deploy the models — and for them to help connect the dots between data and business teams.
Granularity is crucial for effective budget allocation. While MMMs help with high-level spending decisions, lower-level optimization still requires campaign-specific data with performance granularity for each ad type. The biggest challenge is data consistency, as more structured time-series data improves an MMM’s results.
— Ronan Shields contributed to this story
More in Marketing
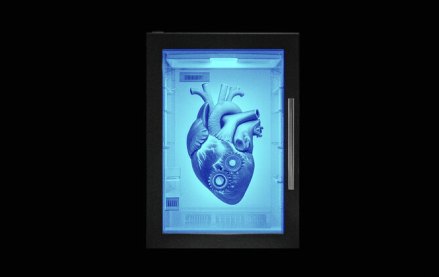
Generative AI sparks brand safety concerns marketers know all too well
Despite concerns around brand misuse and IP, most marketers are sticking to traditional strategies.
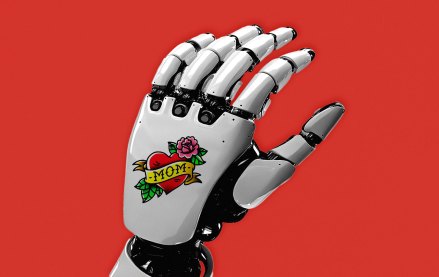
‘Production is a big topic right now’: With AI moving beyond media, Publicis turns toward creative
The holdco is positioning AI as core infrastructure for ad production not just media buys.
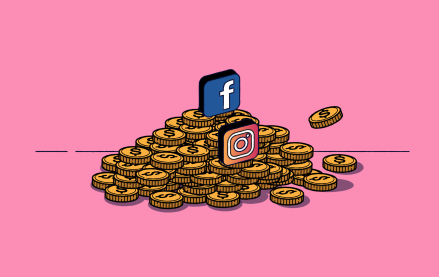
Instagram offers a new guide to advertisers to convince them to try out its creator marketplace
The 26-page document aims to make the whole process quick and painless.