One of the most pressing and unavoidable topics for publishers and advertisers today is finding a way to rebuild the digital ad ecosystem without relying on third-party cookies. In that quest, a whole bunch of workarounds and alternate tech has materialized.
Following in the footsteps of tougher browsers like Safari, Firefox and Brave, as well as tighter privacy regulations in Europe and California, Google is working on alternatives to tracking an identity to maintain a thriving ad-funded web. A key development that has the industry vexed is what will happen when Chrome, which accounts for 65% of global browser share, according to StatCounter Global Stats, limits third-party cookie use in February.
One key alternative it’s working on is federated learning of cohorts, which is a way for browsers to continue allowing interest-based advertising on the web: Instead of observing the browsing behavior of individuals, companies observe the behavior of a cohort (or “flock”) of similar people. Here’s a primer on what to know.
First, what are we talking about here?
More broadly, federated learning uses machine learning to build a robust model without sharing personally identifiable data, a positive step forward given that everyone agrees privacy is a hot-button issue at the moment.
At a high level, the system uses machine learning to train an algorithm across multiple decentralized devices without sharing or exchanging the data from those devices, that data remains stored locally. This makes it much more privacy-compliant. It differs from other centralized machine-learning systems where all data is uploaded to one server. It’s also different from distributed learning in a few ways; for instance, distributed learning assumes all the data sets are identical. In federated learning systems, the data can vary hugely.
So how does Google plan to use federated learning? And why now?
A few months ago, Google set out proposals for federated learning of cohorts, which uses machine learning algorithms that run on the device to group people together into audience interests based on behavior like browser history. Through self-learning, the model builds and becomes more robust, with the flocks representing groups of thousands of people rather than individuals, so is deemed more privacy compliant. This model can then be used to let agencies and advertisers identify the optimal audience segment that are more likely to engage with an ad for a finance or luxury client, for instance.
Makes sense. What’s new about this?
These systems have been around for a while for non-ad related purposes. One of the earlier examples of FLoC frameworks came from Google’s Keyboard, Gboard, to train its smartphone keyboards to use predictive text. Privacy regulations meant it was impossible for Google to upload all the input text data from people’s phones to its own server to train the algorithm to guess the right words. The amount of data that the system would suck up from users’ phones would be prohibitive too. Facebook uses a different but similar machine learning technique, called self-supervised learning, for improving its apps and also in its publisher and advertiser products. The main benefit is it uses privacy by design.
Sounds great. What’s the catch?
There are a few. Google has been getting feedback from across the ad industry about how some of its proposals around developing a privacy-first ad-funded web. But critics are wary of letting Google hold the keys to the artificial intelligence model that it created. Google’s FLoC has come under fire for potentially allowing bad actors to still access sensitive data. Each browser’s flock name identifies it as a type of web user, shared in the HTTP header, which is shared with everyone they interact with on the web.
What about other industries?
There will be more federated learning systems to come. There are applications in industries beyond advertising, including defense, telecommunications and healthcare. Applications are being explored for training self-driving cars, as federated learning would limit the high volume of data that needs to be transferred (in more traditional cloud-based machine learning) and speed up the process.
Update: An earlier version of this article stated that Facebook uses federated learning, rather than self-supervised learning which is a similar but different technique.
More in Media
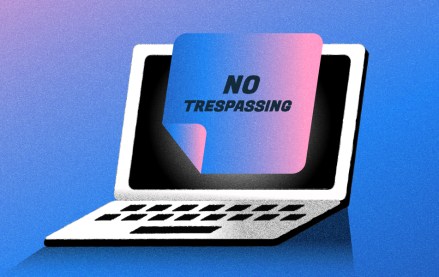
AI Briefing: Senators propose new regulations for privacy, transparency and copyright protections
A new bill called the COPIED Act aims to pass new transparency standards to protect IP and guard against AI-generated misinformation.

Media Briefing: Publishers reflect on ad revenue midway through 2024
Some publishers say ad revenue is pacing 15% up year over year while others are still managing their expectations for how 2024 will shake out.
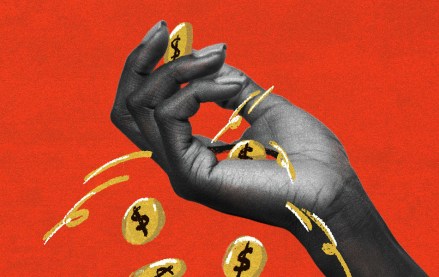
Teads is exploring sale options as M&A in ad tech heats up
Sources state the Altice-owned stalwart of outstream video has recently held talks with private equity and strategic players.