Connect with execs from The New York Times, TIME, Dotdash Meredith and many more
This startup is creating an AI training data marketplace to help creators and companies buy and sell licensed content
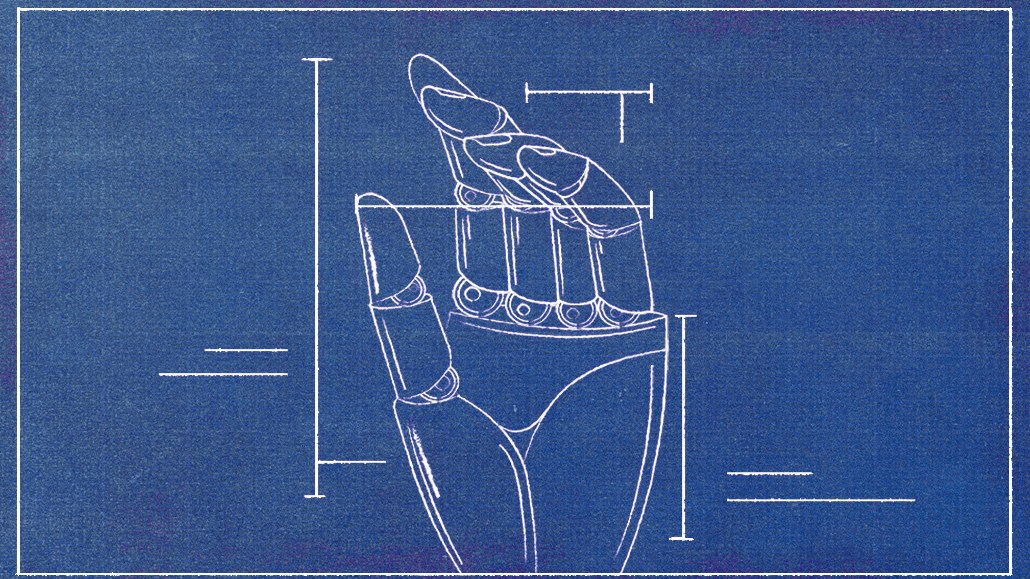
What’s the value of data used in training AI? That’s an existential question one new startup wants to help answer.
Trainspot is launching an AI data marketplace to help content creators monetize their intellectual property for AI training while giving developers and businesses a way to source licensed training data. The San Francisco-based company, which emerged from stealth mode yesterday, aims to attract a range of creators to sell books, images, video and code from writers, filmmakers and developers.
Companies curious about using AI are also cautious about gray areas like the legality, reliability and explainability of AI outputs. Trainspot’s goal is to help with all three for training foundation models, fine-tuning and for improving accuracy with techniques like retrieval augmented generation (RAG).
How Trainspot works
In an interview Trainspot co-founders Ron Palmeri and David Temkin told Digiday the two-sided marketplace has features for both buyers and sellers. Creators can set up a profile and choose to set a price for their content, let it be used for free or block AI models from using it. Each user chooses categories and subcategories for content formats and topics. They also can add other information as metadata to help with discoverability. Trainspot will verify a creator’s account before allowing them to sell, donate or block content.
To buy data sets on Trainspot, companies can filter based on factors like content format, licensing terms, and topics. After selecting, an e-commerce style checkout powered by Stripe will process the purchase. Prices set creators can be updated at any time.
Trainspot’s co-founders have plenty of analogies to explain what they think the marketplace could look like. They say it’s the Spotify equivalent for training data after the Napster era. Or it’s like eBay when it comes to a two-sided marketplace where goods are easily sold and bought. Trainspot aims to help with training data pricing just as Zillow provides market-driven housing estimates. They also hope to offer a catalog of training data just like Hugging Face offers with open-source code.
Many of the AI data deals that have happened so far have been large scale and often opaque without terms disclosed, according to Temkin.
“When it comes to what data is worth, one of the most fascinating things about this whole market is we don’t really know,” Temkin said. “Without an open and transparent marketplace, it’s not clear what anything’s worth. And by creating this product and this kind of a framework, we’re going to be getting away from the current state of the state.”
Temkin and Parmeri have experience with creating and introducing early products in new industries. Temkin previously led the development of Google’s My Ad Center and before that was Brave’s chief product officer of Brave, where he helped scale the privacy-focused internet browser. Palmeri has complementary experience as co-founder of the visual AI firm Skylabs and as co-founder of the early social analytics firm Scout Labs. He also has venture capital experience at places like Minor Ventures, which backed GrandCentral before it became Google Voice.
The emergence of AI models has sparked debate about the economic value of training data – some observer note that data used for training foundation models has a different value than data for grounding AI answers. Industry standards for data pricing and creator compensation are still evolving, with platforms like Shutterstock, Adobe, Picsart and Bria AI exploring various payout models. Other companies like the AI music startup Rightsify have taken to forming trade groups that promote ethically sourced data.
Marketing and tech experts see the need for a platform like Trainspot to help companies source additional data for AI applications. However, there’s also the classic chicken-and-egg challenge that many types of new tech often face. Will the scale of commercially viable data draw more companies to pay for it on the platform? Or will interest from a range of buyers attract more interest from potential sellers?
The first priority for scaling is supplying the marketplace with enough source training data before focusing on increasing demand, Palmeri and Temkin said. For starters, there will be a trove of publicly available content on day zero that is free and pre-licensed. Trainspot also wants to let creators to upload their content from platforms like YouTube and GitHub but they can also upload it directly. As data from content becomes a key differentiator for AI models, the hope is for content creators with large audiences or built-in communities to also spread the word.
“It really does require a critical mass of people that fall into these different categories — whether they’re book authors or YouTubers or people that have websites — to understand this is an action they can take,” Palmeri said. “It’s an action that could help protect them and establish their rights, but it’s also a way for them to participate in the opportunity.”
The platform seems to have potential to empower content creators and address the growing demand for high-quality training data, said Gartner analyst Andrew Frank. Although Trainspot aims to make the platform easy to use, he also noted a low-friction approach might not be best when vetting data for AI. That’s because verifying the quality of data will be as important as verifying the data’s owner.
Frank suggested that the success of Trainspot hinges on establishing a “branded trust” for content, similar to the credibility associated with reputable news publications. He emphasized the need for mechanisms that maintain this trust throughout the AI training process, enabling developers to trace the origins and assess the reliability of training data. He also expressed curiosity about how Trainspot’s model will evolve, acknowledging both the potential and the significant challenges ahead.
“You could see it as a branding problem,” Frank said. “People trust goods and services by their brand. I might recognize a brand and therefore buy it even though it might cost more than a generic version. We need the same sort of market integrity attestation for content … I’m more likely to trust an article from the Wall Street Journal than I am to trust it from an unknown person posting on X.”
Seeing and scaling opportunity
It can be hard determining fair data prices, said Soren Larson, co-founder of Crosshatch, a startup creating an identity layer for user personalization. That’s because the true value of data for specific AI applications is often hidden from sellers, leading to pricing disparities. Larson mentioned strategic pricing tactics, like those used by hedge funds, can further distort the market.
A limited number of buyers and lack of transparency exacerbate these issues, according to Larson. He suggests vertical integration – where data providers directly create value through services – may be a more viable approach than relying on data marketplaces. Pitching a way for creators to get their “fair share” requires also asking about the definition of “fair share.” Another question is whether terms are a one-time deal or something that’s renewed over time. For example, compensating a news company when someone clicks on a link might be easier than earlier parts in the AI training process.
“The pathway to value from either training or fine-tuning is much harder to calculate because it’s a function of how the model ends up being used and how that usage ends up driving value, which itself is just complicated to calculate,” Larson said.
Others see a lot of value in the role an AI data marketplace could play when it comes to improving attribution with AI models. Nikolaos Vasiloglou, vp of research ML at RelationalAI, noted companies are running out of high quality data and face limits when it comes to using synthetic data. Like Larson, he said pricing products in new markets can be a challenge, but added the first step is making data available so that, over time, it will demonstrate value. He thinks Trainspot might want to consider YouTube’s early growth strategy, which focused on consumer-generated content before seeking licensing content from major studios.
“We have a missing spot on the market for this, but maybe the timing might not be right now,” Vasiloglou said. “Maybe we haven’t yet hit the point where companies have such a big adoption of language models that they’re craving for new [data]. So that’s the biggest risk.”
More in Media
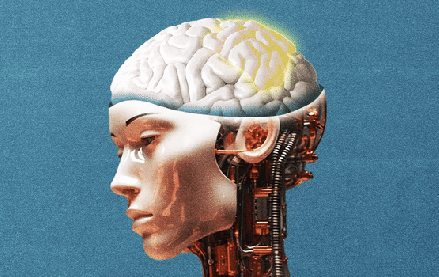
YouTube’s AI slop crackdown has creators concerned, marketers cheering
Despite the potential crackdown, both creators and marketers broadly view YouTube’s updated policies as a positive move. They believe it indicates that the platform is paying attention to the ways creators are using AI — and that it’s open to AI tools that don’t result in the propagation of so-called “AI slop” videos.

Jargon buster: The key terms to know on AI bot traffic and monetization
Here’s a breakdown of the emerging vocabulary of AI-media economics, what these terms mean, and why they matter now.
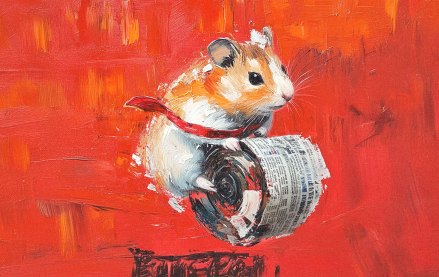
Digiday+ Research: Publishers identify the top trends among Gen Z readers
Gen Z makes up a very small percentage of publishers’ readership, but those Gen Z audience members are consuming their news anytime, anywhere.