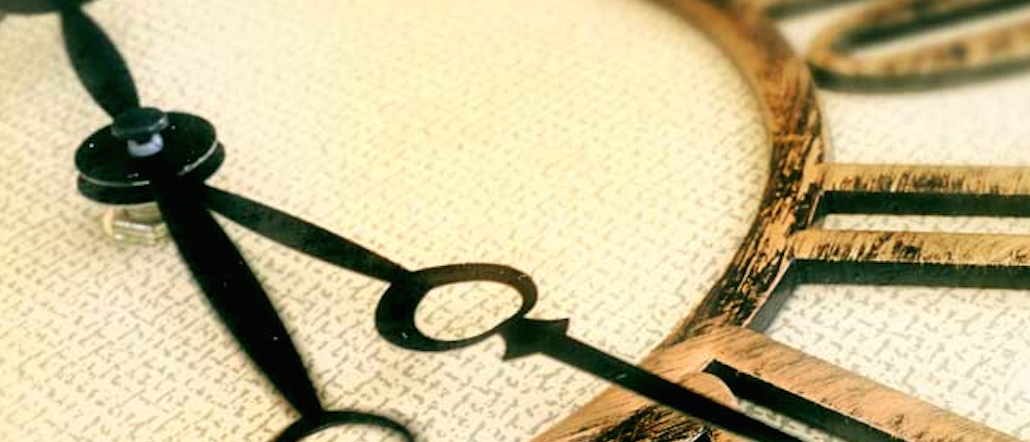
The iPhone–which is turning ten this month– transformed the way people communicate, shop, and travel. It also transformed the way marketers reach their ideal audiences. For the first time, they could use the smartphone’s location data to find out where consumers were—and buy impressions accordingly.
Today, advertisers continue to use real-time location data to target consumers. But the industry has come a long way since 2007. Advertisers are moving beyond the real-time capabilities of location technology towards more advanced applications. Brands can boost campaign performance and ROI, by using historical data and AI-powered solutions to discover where consumers have been and where they are going.
Back to the Basics, Location 101
Location-based advertising means using real-time location data to reach out to consumers based on where they go. A common example of Location 101 is targeting consumers based on their distance from a given location in real-time, known as proximity targeting. For example, if a pharmacy wants to target young women inclined to purchase its products, they can collect mobile devices seen in similar or related locations. Using location technology, the pharmacy brand can retarget that audience group whenever they come within feet of one of the pharmacies with products they have in stock or specific promotions. The same example applies to many verticals, not just CPG.
But even proximity targeting has matured beyond these basic abilities. More advanced applications involve layering real-time location data with other valuable data for more granular targeting.
Let’s say a high-end retailer on a busy shopping street is using proximity targeting to reach consumers within 100 feet of their store. That brand could be wasting impressions on people who wouldn’t shop at a luxury store in the first place. But how would they know? If the brand pairs its proximity data with consumer information such as shopping histories, demographic and geographic details, they can more accurately target the right person. For instance, they can improve campaign performance by reaching out only to high-income earners who have previously shopped in a different luxury store—individuals who are not just nearby, but also likely to buy.
From Mobile to Mobility
Brands need to remember that they aren’t advertising to a mobile device, rather, they are advertising to a mobile person. Therefore, the importance of looking at historical location data to get to know their consumers and their preferences is paramount. For instance, sports enthusiasts could be identified by the quantity of trips they make to sports fields each month. Their habits can be identified by the types of hotels they stay at while on the road to the games. Based on this historical information, advertisers can utilize this data to target this audience with ads for fan gear when close to sporting goods stores or hotel deals when traveling on the road to games. This type of data builds rich audience targeting segments for marketers so they can advertise based on consumer behavior.
Places we go over time reveal more than just consumers’ preferences; historical location data can also tell advertisers things like when consumers tend to go to the gym, how often they go shopping, or how frequently they are heading to the airport—from specific location, the day of week, time, to duration of time spent in a location. Such insights can be used to retarget consumers not only at the right place, but also at the right time.
For example, someone who visits the airport monthly is likely a frequent flyer, but that doesn’t mean they’ll be receptive to an airline ad while boarding a competitor’s plane. Instead of using proximity targeting to reach this flyer at the airport, the airline can analyze historical location patterns to reach out after their travel when they are back at work or at home—and before they book their next flight.
AI and Advanced Analytics
While many brands focus on real-time location and historical behavioral patterns, other advertisers are looking into the future. With machine learning and predictive analytics, these advertisers lean on their technology partners’ knowledge of behavioral patterns and advanced algorithms to drive in-store foot traffic.
Brands drive foot traffic by targeting only those with strong purchase intent at exactly the right time and place. Brands can now tell their tech partners how many visitors they need in what locations and buy them on a cost-per-visit model; only being charged once a store visit occurs.
For example, a fast-food chain can identify its ideal audience by looking at individuals who visit this chain a few times a month. Then, they can deliver ads to these individuals at the time and place they are most receptive based on past behavior patterns to drive them in store. Such advanced techniques increase foot traffic as well as in-store sales.
From basic applications to more advanced use cases, location data and technology continue to alter the advertising world. As the industry evolves, brands should look beyond where consumers are in the moment, to where they’ve been and where they’re going in order to improve conversation rates, waste less ad dollars, and increase sales.
More from Digiday
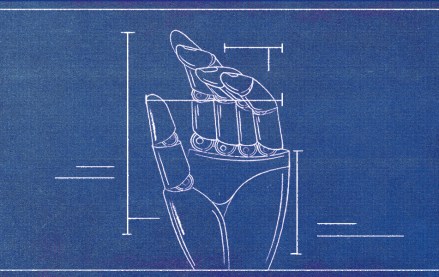
Agencies create specialist units to help marketers’ solve for AI search gatekeepers
Wpromote, Kepler and Jellyfish practices aim to illuminate impact of black box LLMs’ understanding of brands search and social efforts.
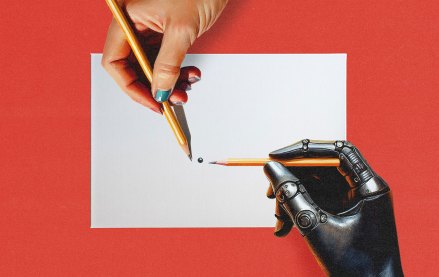
What AI startup Cluely gets — and ad tech forgets — about attention
Cluely launched a narrative before it launched a tool. And somehow, it’s working.
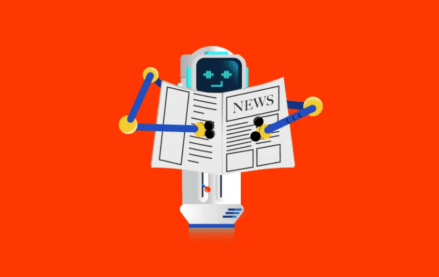
Media Briefing: ‘Cloudflare is locking the door’: Publishers celebrate victory against AI bot crawlers
After years of miserably watching their content get ransacked for free by millions of unidentified AI bot crawlers, publishers were finally thrown a viable lifeline.