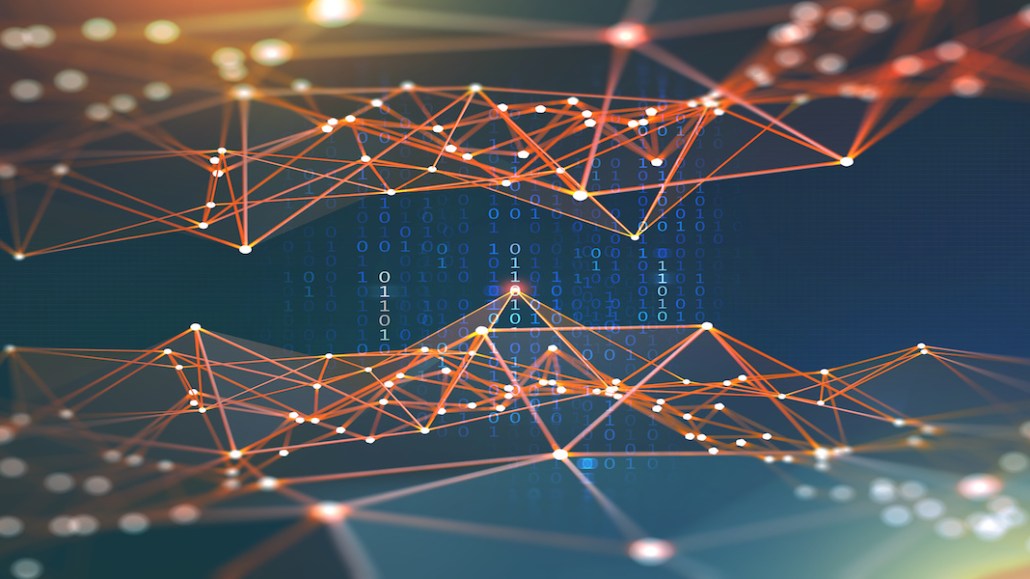
By Oliver Brdiczka, AI and machine learning architect, Adobe
These four principles form the building blocks of a successful relationship between humans and AI.
Artificial intelligence (AI) is powering more and more services and devices that we use on a daily basis, such as personal voice assistants, movie recommendation services and driving assistance systems. And while AI has become a lot more sophisticated, we all have those moments where we wonder: “Why did I get this weird recommendation?” or “Why did the assistant do this?” Often after a restart and some trial and error, we get our AI systems back on track, but we never completely and blindly trust our AI-powered future.
One of the reasons for this distrust is that most current AI systems operate as a ‘black box’ with limited interaction capabilities, human context understanding and explanations. These limitations have inspired the call for a new phase of AI, which will create a more collaborative partnership between humans and machines. Dubbed “Contextual AI,” this new technology is already getting multi-billion dollar investments. Contextual AI is technology that is embedded, understands human context and is capable of interacting with humans.
Let’s explore how Contextual AI works, how it compares to previous phases of AI, the challenges we need to overcome and the progress we’re making at Adobe.
Contextual Artificial Intelligence: The building blocks of a successful relationship between humans and AI
Contextual AI does not refer to a specific algorithm or machine learning method — instead, it takes a human-centric view and approach to AI. The core is the definition of a set of requirements that enable a symbiotic relationship between AI and humans. Contextual AI needs to be intelligible, adaptive, customizable and controllable, as well as context-aware. Here’s what that looks like in the real world:
- Intelligibility in AI refers to the requirement that a system needs to be able to explain itself, to represent to its users what it knows, how it knows it and what it is doing about it. Intelligibility is required for trust in AI systems.
- Adaptivity refers to the ability of an AI system, when trained or conceived for a specific situation or environment, to be adaptive enough, so it can be run similarly in a different situation or environment and meet the user’s expectations. For example, a smart home assistant that controls my house knows my preferences, but will it be able to translate those to my mom’s home when I visit?
- An AI system must be adaptable and customizable by the user. And the user must be able to gain and maintain equal control over all functions of the system. Obviously, this goes together with intelligibility because the user needs to understand the basis of the system’s decisions.
- Finally, context-awareness is a core requirement referring to the capacity of the system to ‘see’ at the same level as a human does, i.e. that it has sufficient perception of the user’s environment, situation, and context to reason properly. A smart home assistant cannot learn my behavior and preferences and control my house with only one camera on the front porch as input.
While true Contextual AI doesn’t exist yet, we are getting closer to it. Self-driving cars are a good example: they are a first attempt to understand more of the human context (in this case the road, the state of passengers, or dangerous situations). However, the current understanding is still very limited and narrow. In the 1980s TV series Knight Rider, for example, the car (KITT) demonstrates the principles of true Contextual AI, as it was able to interact seamlessly with the driver, understand everything that was going on and help in dangerous situations. Obviously, it was far-fetched and fictional, but the essence is that Contextual AI needs to have a deeper understanding of a human’s situation and be able to interact and explain itself.
What differentiates Contextual AI from previous phases of AI?
Contextual AI addresses many of the shortcomings of previous AI developments or phases. Historically, AI started as handcrafted knowledge. This rule-based AI had no learning capability and was mostly designed by engineers. Think of chess computers (remember when Deep Blue beat Garry Kasparov?) or expert systems. They had their first successful applications from the 1980s to the early 2000s. However, as a machine doesn’t have the same perception as a human, it fell short when a clear specification of the rules, in particular for sensor signal input (audio and video), wasn’t possible.
Statistical learning, particularly deep learning, addressed some of these shortcomings by inferring statistical patterns (that a human might not see or know) from very large datasets and raw signals. This led to the recent success of AI in image recognition, voice, conversational interfaces and many more applications. However, large scale statistical training has downsides as well. For one, statistical models such as deep learning models can be easily attacked or confused. Adversarial examples can be generated and tuned to make a production-grade machine learning system. Minor changes to the pixels in the input image, barely visible to the human eye, can yield very different recognition results. You can even generate your own adversarial examples to fool the algorithm. Additionally, as most AI approaches rely on large-scale data, unconscious bias can creep into AI algorithms based on the (positive and negative) examples with which they’ve been trained.
While the hype around AI is still powered by statistical learning, leading researchers have started questioning the real “intelligence” of the industry’s current AI approaches. While statistical algorithms helped with the context-awareness and adaptivity that is needed for a Contextual AI system, they do fall short on the requirements for humans to understand what is going on, and to customize and control it. A ‘black box’ algorithm cannot be trusted in critical situations. It’s unclear what structures the statistical AI algorithms really learn and whether the algorithms just separate data examples or have a true understanding of the content.
Achieving a deeper understanding of human-machine interactions
We’ve come a long way in the journey towards true Contextual AI. We now understand human-level concepts in images and AI can more naturally interact with the human using these concepts. However, we still require a deeper understanding of language as well as new human-computer interaction paradigms. How should an AI system and humans interact in the future, for example? Through voice, gestures, or even implants?
More importantly, the representation and recognition of what humans think and do is still very limited. For example, millions of creatives use Adobe’s products every day and while we’re familiar with how they’re using our tools as part of their work, we are still working toward fully representing “creative intent.” What does the creative user want to do? What are the steps in the process? And what may he or she require for success? And how could a user even teach an AI system what his or her creative intent is?
Some future technical directions that are currently being explored are explainable AI models and common sense reasoning. How could we teach the common sense of a five-year-old to an AI system? And how could we further make it explain itself and make it fully contextual? At Adobe, we believe that AI enhances human creativity and intelligence (AI doesn’t replace it) when it comes to designing, optimizing and delivering digital experiences. Therefore, it is important to leverage the power of Contextual AI to help move the industry forward and harness its power to continually innovate.
More from Digiday
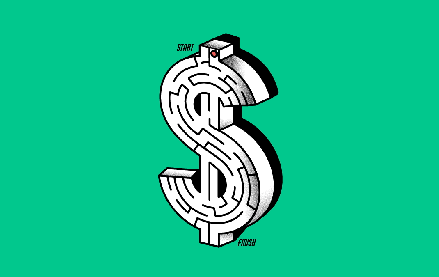
Key takeaways from Digiday’s 2024 Gaming Advertising Forum
Now that gaming has gone from a buzzword to a regular presence in brands’ media mix, marketers are more closely scrutinizing the value and ROI of their investments in this channel — and the platforms are rising to the challenge. Here are some of the biggest takeaways from this week’s Gaming Advertising Forum.

Media Briefing: Publishers’ Q4 programmatic ad businesses are in limbo
This week’s Media Briefing looks at how publishers in the U.S. and Europe have seen programmatic ad sales on the open market slow in the fourth quarter while they’ve picked up in the private marketplace.
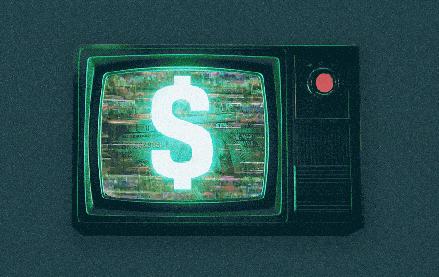
Queries mount as The Trade Desk takes an unprecedented step into TV’s adland
Industry peers want to now more about the DSP’s trading deals and broader GTM strategy as it heralds greater CTV efficiencies.