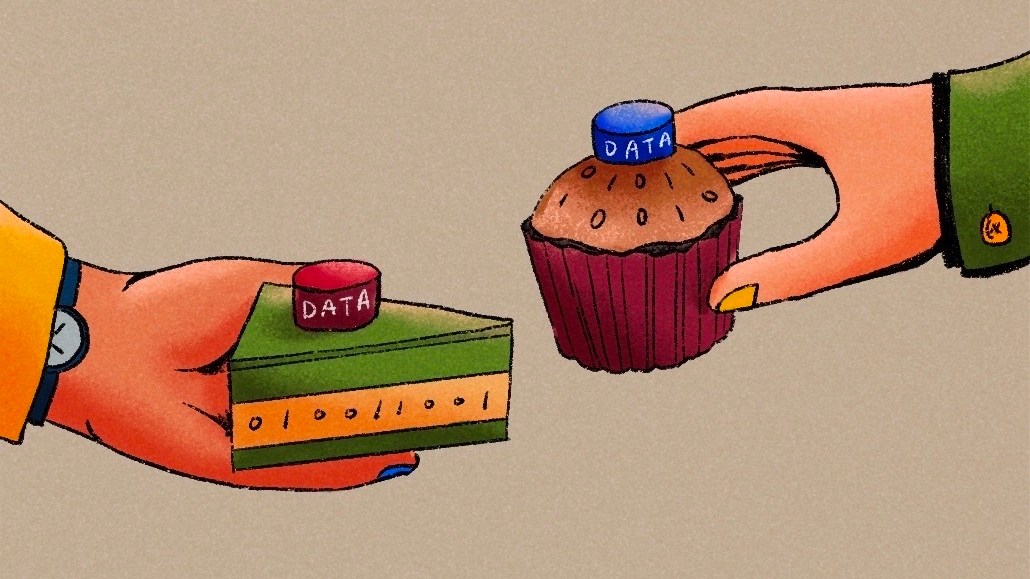
This article is a WTF explainer, in which we break down media and marketing’s most confusing terms. More from the series →
Originally published on April, 10, 2019, this article has been updated to include an explainer video.
As the ad industry re-evaluates its approach to personal privacy, advertisers are searching for ways to collect data on people without compromising their privacy. One of those alternatives has been called differential privacy, a statistical technique which allows companies to share aggregate data about user habits while protecting individual privacy.
Here’s an explainer on how differential privacy works.
WTF is differential privacy?
It’s a process used to aggregate data that was pioneered by Microsoft and is now used by Apple, Google and other big tech companies. In a nutshell, a differential privacy algorithm injects random data into a data set to protect individual privacy.
Before data is sent to a server to be anonymized, the differential privacy algorithm adds random data into an original data set. The inclusion of the random data means the advertiser gets a data set that has been masked ever so slightly and, therefore, isn’t quite exact.
How so?
The advertiser effectively gets approximations of the answers they need without compromising anyone’s privacy. An advertiser viewing differential privacy data might know that 150 out of 200 people saw a Facebook ad and clicked through to its site, but not which 150 people, for example. It gives the users of that data plausible deniability because it’s virtually impossible to identify specific individuals with full certainty.
That doesn’t sound very accurate.
There is a definite trade-off here between privacy and accuracy as advertisers won’t get the full picture of how people respond to a campaign. However, it’s a sacrifice some advertisers seem willing to accept. Without the random data injected into the main data set, it’s easy to figure out who the person who engaged with the ad is, which would mean having to kill the database if the proper General Data Protection Regulation consent has not been attained.
Who is driving this?
There is a Truth in Measurement cross-industry collective of advertisers, publishers and tech platforms considering how the statistical technique could be used to underpin cross-platform measurement. Trace Rutland, director of media innovation for Tyson Foods, who is part of the collective, said this pragmatism comes down to there being a more apparent ethics test at play that revolves around the question: “Would our customers expect and be comfortable with us using their data this way?” The answer to which pushed the cross-industry collective to consider whether differential privacy could be used as a way to validate data being shared in a proposed data clean room.
How can that help with cross-platform measurement?
With all the talk of whether data clean rooms can support cross-party measurement, one sticking point has been who actually benefits from it. Media sellers are wary of sharing their data in the same place as their rivals, while advertisers don’t feel like they have ownership of those environments, which subsequently makes them suspicious of what’s been added.
Differential privacy could ease some of those suspicions as all backers of the clean room would feel like they have some control of a data anonymization process that is usually controlled by the media seller. An advertiser would get a data set that is an accurate reflection of how well a campaign performed, while the media seller wouldn’t have to part with valuable targeting data.
The issue came up at an event hosted by Truth in Measurement group last month. “The consensus was that advertisers would receive a differential privacy-based log file of campaign data as an output of data clean rooms being adopted,” said Victor Wong, CEO of Thunder Experience Cloud, which has spearheaded the Truth in Measurement initiative.
Can any advertiser do this?
Any advertiser could theoretically develop their own algorithm for differential privacy, but it’s not advisable given how complex it would be to develop and then manage. Indeed, advertisers like Tyson Foods would rather work with others to co-fund a version of the technique they can apply to larger data sets.
“If something like differential privacy is going to take off, then it needs to be a combined effort on the buy side. Advertisers can’t do this alone,” said Rutland, who wants the industry to rally around a united version of the algorithm rather than support various versions of it. “Whenever advertisers have tried to go it alone when it comes to cross-platform measurement, it’s not been something they’ve been able to scale to a point where it’s had an impact on the way the walled gardens go to market.”
Any other downsides?
Differential privacy isn’t great on small data sets. The smaller the data set, the more prone it is to inaccuracies once the random data is added to it. Furthermore, it’s harder to make differential privacy work at scale compared to reporting the real, anonymized data of users.
More in Media
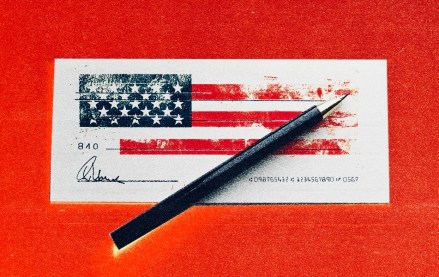
Biggest creator lessons from the 2024 election: podcast showdown, TikTok trends and news influencers
This political cycle, election campaigns increasingly integrated influencer strategies, particularly through long-form podcasts on YouTube and Spotify and short-form content on TikTok.
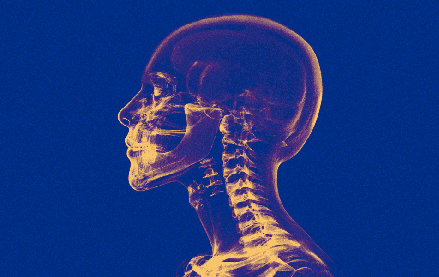
AI Briefing: Inside Accenture and Nvidia’s plan to scale AI agents for enterprise business
Accenture and Nvidia execs explain how they hope to build and scale generative AI tools for marketing and other business functions.
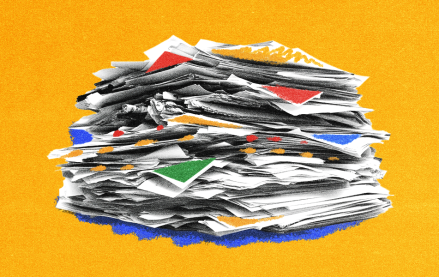
Google’s ad tech empire rests on how well it can make these key arguments
Lawyers will argue the government’s case is outdated, and its innovations don’t mean it’s obligated to share with rivals.