Amazon adds tools for scaling generative AI applications — and improving accuracy issues
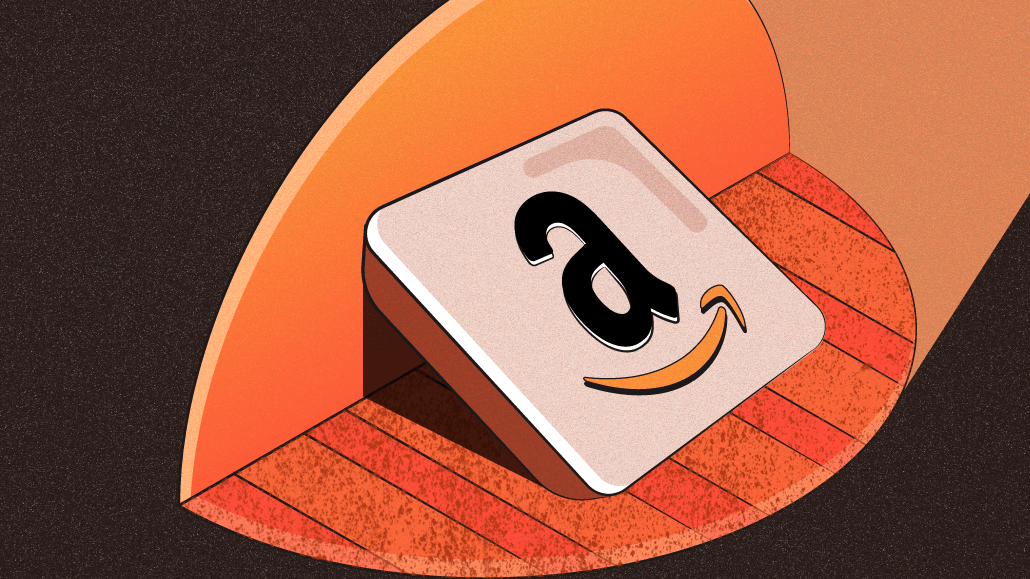
Amazon is adding more ways to make generative AI applications easier to create, more useful to adopt, and potentially more accurate.
Amazon Web Services yesterday used its AWS Summit New York event to announce new ways to make enterprise-grade AI apps while also improving the accuracy of large language models — a key hurdle for attracting companies wary about “hallucination” issues with various LLMs.
One addition is contextual grounding checks, a technique for evaluating AI-generated answers by cross-referencing source material in real time. Because different companies might have different tolerances for accuracy based on their industry and types of data, grounding checks will also measures relevance in order to block answers based on a company’s tolerance level.
Another new feature from AWS is a Guardrails API, which will evaluate user prompt inputs and AI model responses for various LLMs within Amazon Bedrock, or evaluate a company’s own LLM. The API will also help identify content based on a company’s policies to redact sensitive information, filter harmful content and block undesirable topics or inappropriate content.
“An [API] request now can be much more specific and tailored to ensure that it’s the appropriate output, right for that request or that input,” Diya Wynn, responsible AI lead at AWS, told Digiday. “The thing that is important here is it’s allowing customers to have an additional layer or level of safety. And that API provides that to any LLM, not just those that were in Amazon Bedrock.”
In Amazon’s tests, contextual grounding checks found and filtered up to 75% of hallucinations in AI model responses and blocked up to 85% more content when used with the Guardrails API. Onstage at AWS Summit NY, Matt Wood, vice president of AI products at AWS, said AI models trained on the public internet use a “very, very broad” set of data compared to the types of data sets and document formats businesses use.
“That information is usually pretty shallow relative to the depth of information that most organizations deal with day to day,” Wood said. “When you get down into the depth in these world models, they can turn a little bit into Swiss cheese. There are areas of information density, there are areas of information sparsity. And where you have that information density and the models have context, the models do really, really well.”
The updates were just two of many announced at AWS Summit NY, where the e-commerce giant also rolled out other capabilities for its generative AI platforms. Others included the debut of a new AWS App Studio, which aims to let enterprise customers create AI apps from text prompts; and the expansion of Amazon Q Apps, which will let customers build their own AI apps.
Amazon’s efforts offer one example of the various ways AI model providers are racing to find ways to make generative AI tools easier, more helpful and more accurate. This week, the AI startup Writer released new upgrades for its own AI platform. Using a graph-based approach to retrieval augmented generation (RAG), Writer introduced a new way to built RAG into the process to analyze up to 10 million words when developing chat apps. The four-year-old firm also introduced updates to help AI models explain their process for generating answers — a key industry challenge with improving explainable AI — and new “modes” for customers when checking documents depending on different tasks.
Users won’t just naturally trust answers that come out of the black box, explained Deanna Dong, Writer’s head of product marketing. She added that while generative AI may be “magical,” it still isn’t a “magic bullet that solves everything.”
“We’ve seen that one-size-fits-all chat apps with an open-ended prompt don’t always lead to the best outputs for users,” Dong told Digiday. “There’s a lot of confusion, and it relies so heavily on the user to be like experts at prompting.”
Part of the challenge with AI adoption is companies don’t always know what they need or want to build, said Karli DeFilippo, svp of experience at Media.Monks, an AWS agency partner. That requires more examples for what’s possible while also alleviating brands’ fears of what happens if something goes wrong with an AI initiative.
“If we get a brief or something and [a client] not ready, we’re not just going ahead and doing it anyway,” DeFilippo said. “It’s almost like analysis paralysis. They know that they want to get in there, that they’ve been given a KPI, or that their boss is hounding them. But they still seem to need to know exactly what’s right, what choices are right for them, because there are a lot of choices right now.”
More in Media

Media Briefing: Publishers’ Q4 programmatic ad businesses are in limbo
This week’s Media Briefing looks at how publishers in the U.S. and Europe have seen programmatic ad sales on the open market slow in the fourth quarter while they’ve picked up in the private marketplace.
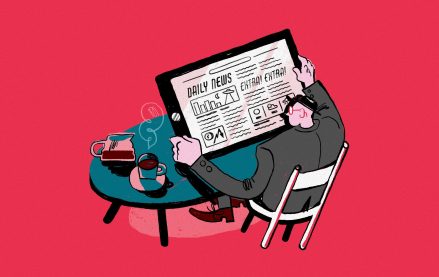
How the European and U.S. publishing landscapes compare and contrast
Publishing executives compared and contrasted the European and U.S. media landscapes and the challenges facing publishers in both regions.
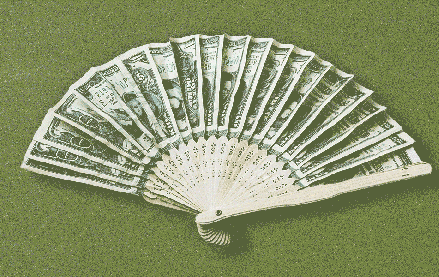
Media Briefing: Publishers’ Q3 earnings show revenue upticks despite election ad pullback
Q3 was a mixed bag for publishers, with some blaming the U.S. presidential election for an ad-spend pullback.