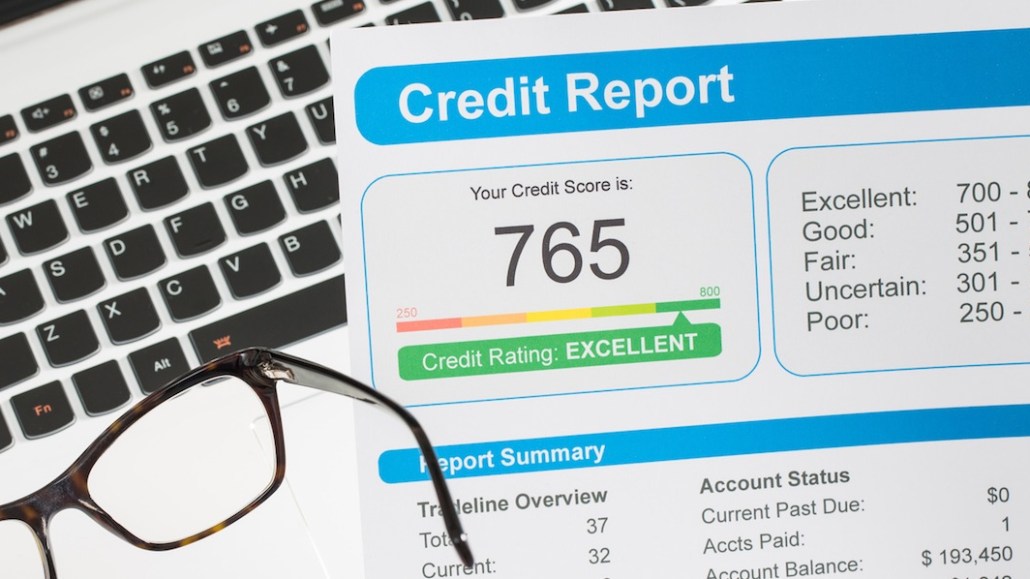
A credit score has a major impact on a person’s life. It’s the key to getting a car loan, a house or an apartment. The traditional way scores are calculated is a method called logistic regression, which means assigning a value to a number of factors in your financial life (for example, payment history, number of credit accounts, length of credit history) and weighing them.
But credit bureaus are now looking into other ways to determine an individual’s credit history beyond the result of a static formula. They’ve integrated machine-learning into credit scoring methods to get a more balanced picture of someone’s likelihood of defaulting on a debt. But it’s more complex than that. Here we break down the method.
The basics
This technology essentially integrates machine-learning into the credit-scoring process. There are multiple tools: NeuroDecision technology is a tool developed by credit bureau Equifax. Other credit bureaus, including Experian, are also reportedly exploring similar ideas. The technology is modeled on neural networks in the human brain, so it can assess the interrelationships between the different factors rather just spit out a number based on a static formula.
“Each attribute can have multiple weights,” said Peter Maynard, svp of enterprise analytics for Equifax. It allows you to better or more accurately predict the person’s likelihood to default.”
The process
Artificial neural networks mimic the way the human brain works, so a non-human has the ability to think through the data and assess patterns.
So the machine has the ability to process the data like a human. Perhaps an individual with a historically poor payback record made improvements over time — a machine-learning tool may be able to take those details into consideration.
“A neural network more closely mimics the way humans think and reason, whereas linear models are more dogmatic — you’re imposing structure on data as opposed to letting the data talk to you,” said Eric VonDohlen, chief analytics officer at the online lender Elevate, in an interview with American Banker.
The traditional method has been popular because of the ability to provide specific explanations to customers, Maynard said. By contrast, neural networks have been seen as a “black box” due to the inability to understand how the decisions were determined. What’s new with Equifax’s tool is the ability to find specific reasons to explain why someone is declined credit. “The algorithm Equifax created allows full transparency into how each consumer is scored,” he said.
The implications
There can be biased outcomes depending on the human that inputs the data. Some industry watchers are concerned that the use of machine learning to analyze financial data can generate biased results. How the data is fed into the system and the instructions that are given to the machine are important factors contributing to biased outcomes, said Kevin Petrasic, partner at international law firm White & Case.
“The program can have the very powerful capability to model itself over time and turn into something it wasn’t originally programmed to do,” he said. “It may come to the conclusion that loans to people in a particular zip code aren’t good credit decisions, or people who hang out in a certain social network are not good credit risks because of whatever associations they have with people in that network.”
Equifax doesn’t share these concerns — for now. Maynard said its product was vetted to meet regulators’ standards, including those of the through regulators including the Office of the Comptroller of the Currency, the Federal Reserve and the Consumer Financial Protection Bureau.
“Currently, we do not have concerns, said Maynard. “First, the sample of data used to build the model is algorithm agnostic, meaning we use the same sample of data for both logistic regression and neural nets. Second, we follow our rigorous compliance and model governance processes to review and approve the model for use.”
More in Marketing
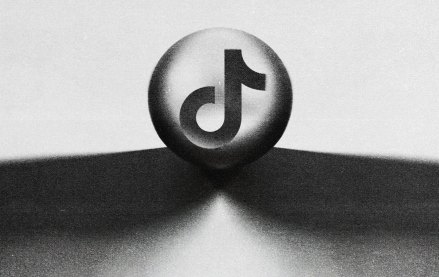
Marketing in limbo: the long shadow of TikTok’s turbulence
When TikTok stumbled, marketers scrambled.
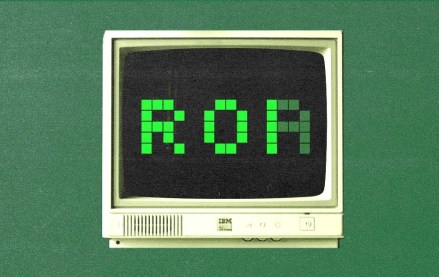
ROAS vs. incrementality: Scrutiny around ad spend grows in booming retail media space
In the fast-growing channel that is retail media, marketers are prioritizing incrementality over ROAS to justify ad spend.
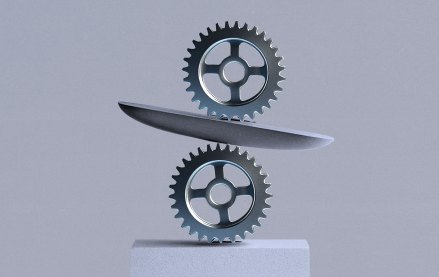
WTF are AI agents?
Despite so much use of the A-word, it’s still early for AI agent adoption, meaning marketers should ask what agents are for, how they’re made, what they do, what they might do — and what they can’t do — including potential reputational risks.