In my last post, I noted that while Big Data is certainly exciting and real, we have been here before, and, in particular, there are some lessons from the earlier encounters that might be handy to bear in mind. Surely, our ability to work with data has changed a lot. The machinery is a lot more powerful, there are more software frameworks, the stockpile of algorithms is much bigger. Still, some fundamentals have not changed in the slightest.
The first important lesson is called the Curse of Dimensionality (CoD) and it somewhat undermines the power of the word ‘big’. What exactly do we mean when we say Big Data? Big as compared to what? Surely, there is much more data in the world. It is hard not to be impressed by how much data is generated on the web or how much data is going to be generated by embedded devices. These datasets are orders and orders of magnitude bigger than the wee little datasets people see in their first statistics classes.
As with many phenomena in science, intuition can be a very suspect guide. The reality is that the right way to think about the size of data is with respect to the dimensions of the problem you are trying to solve. There has been a lot of very technical work done in this area, but the purpose of this short post is not to try to explain those ideas, but just to give a sense of the issue. Because it is NOT just a theoretical issue. It’s as real as it can be.
The basic problem is that as the dimensionality of problems goes up, the amount of data you need to do reliable estimates does not scale linearly. It scales exponentially. This scaling fact (and it’s a fact) means that no matter how much data you think you have, the problem actually demands WAY more data. And not just a little bit more – orders of magnitude more. So the first cautionary tale, is beware your own enchantment with how much data you have and can manipulate. There is a decent chance you do not have enough.
There is no way around CoD except to be careful in how you choose the problems you are trying to solve. That is, you can’t change the fact that data needs can easily outstrip the supply (it never stops being a scarce resource). You CAN, however, sculpt the problem carefully and modestly to make the best use of the data you do have. The natural temptation with Big Data is to let ambitions run wild (it happened a lot in the early days of data mining). Instead of attacking the BIG PROBLEM , try to learn ONE SIMPLE THING. Then iterate and try to learn another simple thing. That is, don’t let Big Data lead you reflexively to Big Problems. Making incremental progress is just fine.
There is this natural tension that I’ve seen many times in organizations that base their business ambitions on the new valuable resource of big data. It’s hard not to be enchanted by the fantastic possibilities that seem just beyond the horizon – Big Data feels like a powerful rocket fuel that will get the ship there fast. This triggers the very deep instincts of technical folks to go chase the big interesting problems as the business folks lick their chops in appreciative anticipation. I’ve seen the story end badly many times.
In the next post, I’ll spend some time talking about some things that I’ve seen work and take some guesses about what problems are likely to yield to the Big Data now piling up at our door like snowdrifts.
More in Media
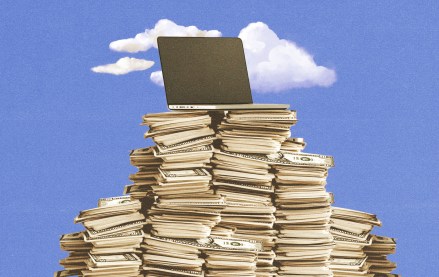
BuzzFeed’s sale of First We Feast seen as a ‘good sign’ for the M&A media market
Investor analysts are describing BuzzFeed’s sale of First We Feast for $82.5 million as a good sign for the media M&A market — which itself is an indication of how ugly that market had become.
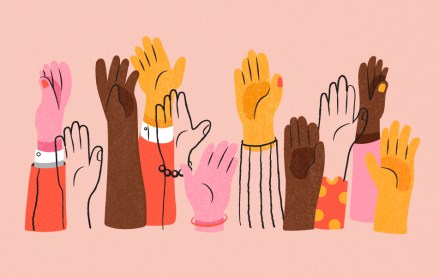
Media Briefing: Efforts to diversify workforces stall for some publishers
A third of the nine publishers that have released workforce demographic reports in the past year haven’t moved the needle on the overall diversity of their companies, according to the annual reports that are tracked by Digiday.
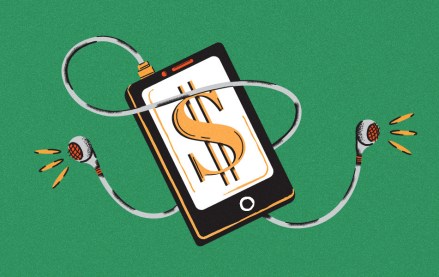
Creators are left wanting more from Spotify’s push to video
The streaming service will have to step up certain features in order to shift people toward video podcasts on its app.