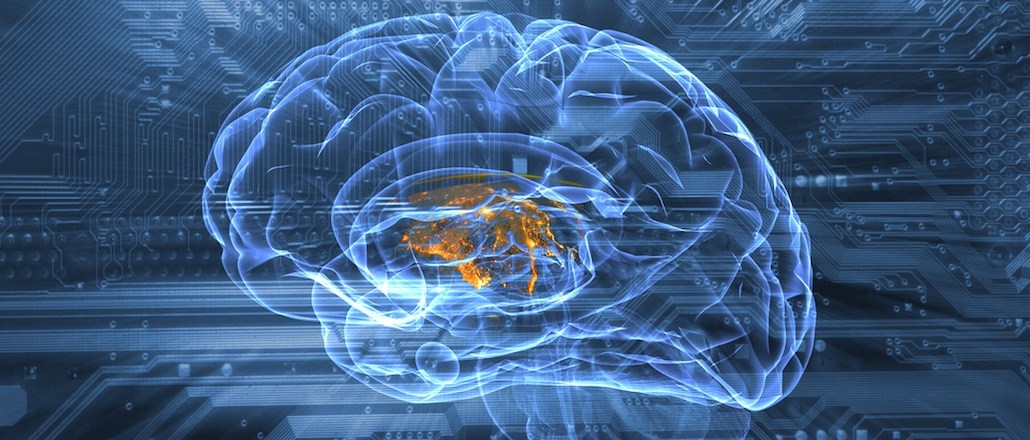
As consumer banking becomes increasingly virtual, banks are setting the bar high for their non-human ambassadors.
Machines will soon be able to carry out many autonomous thinking tasks once performed by humans, including figuring out how to respond to questions on the fly, and analyzing customer data in ways that would have been unheard of just 10 years ago. This is all the result of machine learning, an application of artificial intelligence that lets a computer think and learn on its own.
In January, Canada’s largest bank, the Royal Bank of Canada, announced that it would be working with Richard Sutton, a noted artificial intelligence expert, to explore how it can better integrate machine learning into its work. RBC isn’t alone among major world banks that are relying more heavily on this technology. JPMorgan Chase recently hired a global head of machine learning who came from Microsoft, and last fall, Citibank, through Citi Ventures, made a major investment in Feedzai, a company that uses machine learning technology for fraud prevention.
Sutton is a computer science professor at the University of Alberta and now RBC Research’s head academic adviser in machine learning. He’s been called the father of “reinforcement learning” — an area of machine learning that uses a system of rewards and punishment. RBC will open a machine learning-lab in Edmonton, Alberta, the second if its kind in Canada.
Digiday spoke to Professor Sutton to learn more about how this technology is changing banking.
What is reinforcement learning?
Reinforcement learning goes beyond supervised learning. With supervised learning, you [the machine] have many examples of behavior. There’s no goal except you should behave like the example, but you’re not trying to achieve anything.
Such as?
A typical example is, I show you many pictures, and you learn which ones are cats and which ones are not cats. You as a machine have to guess, and there’s no consequence if you get it right or wrong. It’s about training the machine to make similar distinctions as people make.
Do banks use supervised learning, too?
[Yes.] In situations that involve fraud detection, they can see from examples of those transactions which ones were fraudulent and which ones were not. From those examples and patterns, they can make good guesses for other cases and things that look like they might be fraud and bring them to the attention of people or authorities.
Where does the reinforcement come in?
In real life, you have to learn the mappings of ‘if I do this, what will happen?’ You go down the street; you don’t know what’s down the corner. Life is made up of these sequentially dependent events, and with supervised learning, there are no sequential dependencies. In reinforcement learning, you’ll get a consequence — a reward — and that consequence is visible to the machine.
So you’re punishing robots?
For reinforcement learning, you need three things — a situation, an action and then a reward.
What does that look like in the real world?
They often use reinforcement learning to decide what to put up on web pages — for example, should they put up a particular ad? They often set those up so that the action is putting up the ad, and the reward is the probability of clicking on it, so a good [ad] placement is one that generates clicks. If a machine puts up an ad and no one clicks on it, then the ad is less likely to go up in the future.
How do banks use it?
Let’s say you [the machine] were on the teller side. If the customer hangs up on you, that shows they’re unhappy; it doesn’t tell you what you should have said. It tells you what you did say wasn’t the best. That becomes a reinforcement learning situation. You can learn from trying things until you find one that gets a good outcome.
That sounds a lot like how people act. Why not just use people?
A bank is a big information-handling company. Whether you’re handling information or analyzing it, there are opportunities for optimizing the process using data and machine-learning methods. The vision is that they become more effective at using information and making customers happy. Ultimately, it’s doing things faster by automation, and doing it less expensively.
More in Marketing
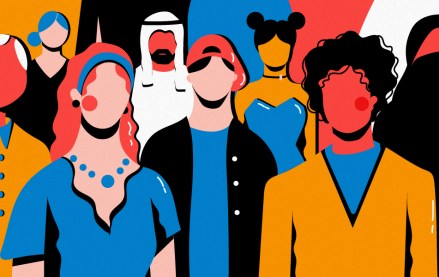
Hyve Group buys the Possible conference, and will add a meeting element to it in the future
Hyve Group, which owns such events as ShopTalk and FinTech Meetup, has agreed to purchase Beyond Ordinary Events, the organizing body behind Possible.
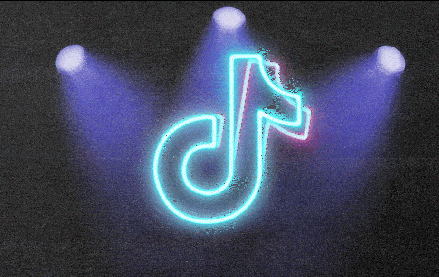
Agencies and marketers point to TikTok in the running to win ‘first real social Olympics’
The video platform is a crucial part of paid social plans this summer, say advertisers and agency execs.
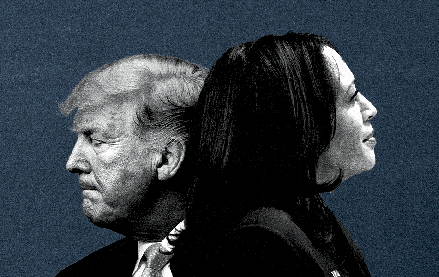
Where Kamala Harris and Donald Trump stand on big tech issues
The next U.S. president is going to have a tough job of reining in social media companies’ dominance and power enough to satisfy lawmakers and users, while still encouraging free speech, privacy and innovation.