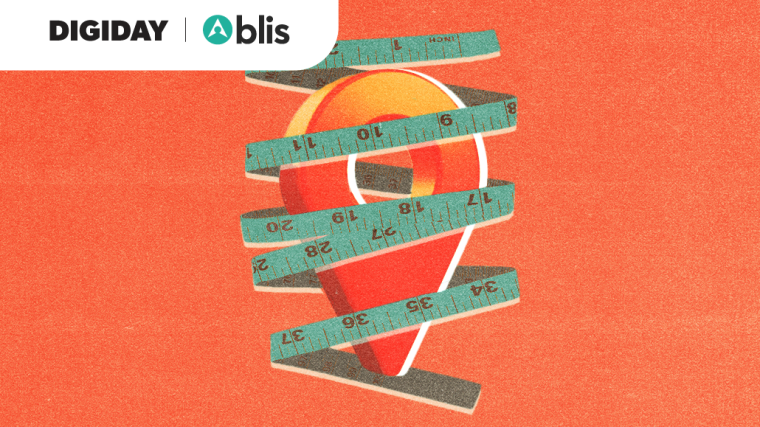
- 01 WTF is geo-based omnichannel measurement?
- 02 What measurement solutions are being explored today, and why is omnichannel measurement so tricky?
- 03 OK, so how does geography factor into better solutions?
- 04 How does a geo-based approach solve the challenges other omnichannel measurement solutions can’t?
- 05 How is this geo approach different from other location-based A/B testing and measurement strategies?
- 06 What other benefits does this omnichannel measurement approach provide?
- 07 How do I select a geo-based omnichannel measurement provider or partner?
This WTF guide, sponsored by Blis, explores how geo-based omnichannel measurement works, what it looks like and its benefits to advertisers.
Marketers need a long-term solution for cross-screen measurement that delivers the results advertisers are accustomed to without getting lost in siloed inefficiencies or proxy metrics. As new programmatic channels emerge and the availability of cookies and personal identifiers decline, the industry faces an urgent challenge: developing an omnichannel measurement framework that addresses these shifts.
Moving away from the dependence on cookies or IDs and letting go of the traditional one-to-one attribution mindset requires connecting all campaign touchpoints, whether an ad is seen on TV at home, on a mobile phone or an out-of-home screen. This new approach must address current limitations while aligning with new consumer behaviors and media consumption habits. Most importantly, it must focus on metrics that truly matter to marketers and their business outcomes.
“In practice, a new measurement framework that doesn’t rely on IDs would allow marketers to focus on the actual business outcomes they’re trying to achieve,” said Amy Fox, Chief Product Officer at Blis. “Not only is this essential for proving true impact, but it’s handy for outlining the validity of the advertising you run and defending requests for increased advertising budgets. Companies don’t care about how many people land on their site. They care about metrics that tie back to business outcomes.”
In this WTF explainer guide, Digiday and Blis dive into geo-based omnichannel measurement, highlighting what it looks like, how it works and the benefits it presents to advertisers.
Countless solutions are floating around, each with its own use cases, benefits and limitations.
Device graphs — data maps that connect users anonymously to their devices — can help connect users across platforms. However, they only effectively measure where there is device graph linkage, leading to a partial and fragmented view. This can give the illusion that reports work as they always have but are very low in resolution, often leading to inaccuracies.
Multi-touch attribution provides granular insights into the effectiveness of a specific marketing channel by assigning credit to multiple touchpoints along the purchase path. While rigorous in its setup, its inability to capture offline touchpoints and heavy reliance on IDs means that MTA can’t fully track a user across all relevant touchpoints and cannot be deployed across more traditional media channels. This presents a fundamental flaw: The last ad a consumer saw isn’t necessarily what drove the behavior.
Media mix modeling, a statistical analysis technique used to assess the impact of different advertising channels, offers a macro-level view of the impact of a company’s marketing mix on its outcomes. However, it usually requires months’ worth of data that runs at broad levels (like DMAs) to build a statistical model, involves complex variables and has a high startup cost, which tends to price out smaller brands. It utilizes probabilistic modeling rather than deterministic and provides a better read on individual channels over time than overall performance by a single campaign. This can be most effective when used in tandem with campaign-specific measurement.
Proprietary ad measurement frameworks like Privacy Sandbox and SKAdNetwork from Google and Apple respectively, are exclusive to each company’s browsers. Due to their siloed framework, there are questions about their scalability. These provide the most narrow and speculative view of performance, lacking full applicability, even across a single channel.
While all these solutions have benefits and a place in the measurement ecosystem with different insights and outcomes, they also have limitations. Another challenge is that many of these methods don’t look beyond traditional means of attribution. With third-party cookies and identifiers declining, a pivot from the heavily relied-on unifying denominator is in order. The way forward requires a measurement approach that operates on a common framework similar to planning and buying — geography, for example.
“We’re at a critical point because online data is becoming more difficult to access and utilize due to restrictions in place, meaning digital is having to look at more traditional methods for planning and measurement,” said Fox. “At the same time, a lot of traditional buying is becoming smarter. We’re at the perfect time to combine traditional and digital advertising and start planning and measuring using a unified data set across a full plan. We believe location could be the key to how we can do that.”
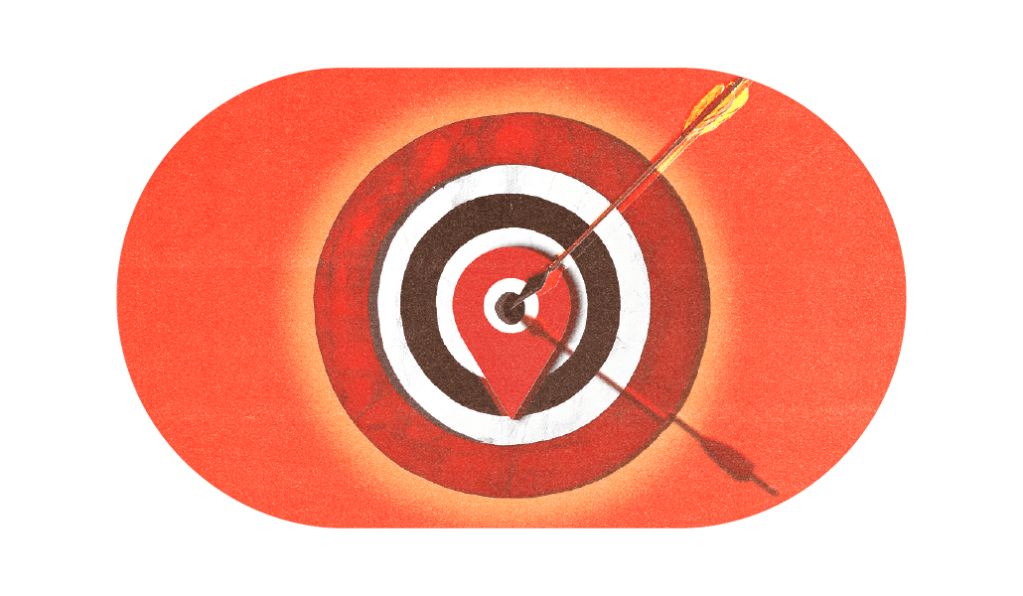
Between third-party cookies being removed from Safari and Firefox, many other types of IDs continue to be restricted, and 80% opt out of app tracking on iOS, so advertisers can’t solely rely on IDs anymore. So, advertisers need to think about planning and buying in a different light.
“If you’re building audiences using IDs and layering them on top of each other, two critical things are limited by that: The first is that the audience is almost entirely running or made up of Android or Chrome identifiers because there are very little amounts of Safari or iOS data still being shared in the industry,” said Fox. “The other is when you’re still dependent on IDs and take a bag of IDs and another bag of IDs, you’ll get big match rate issues between those two. And sometimes the audience size can be hugely compromised by doing that kind of layering.”
This results in a skewed view of the audience and potentially means advertisers only reach half of an audience, especially in the U.S., where Apple and Safari usage is hugely popular.
A standout solution that addresses these concerns and key components is rooted in geography.
“By shifting away from using IDs as the thing that we join and instead using them as a seed to aggregate data at the geo layer, we can better protect consumer identity,” Fox said. “This approach aligns with consumer demands and industry trends and improves match rates for audience planning without compromising delivery across platforms — delivering equally across Android and iOS — as well as channels, like TV, OOH, audio and display.”
A geo-based approach can be a foundational part of the solution because it supplies a common data point across channels. Using geography as a measurement framework isn’t new; however, advancements in machine learning have enabled much smarter, more granular capabilities.
For this new geo-based omnichannel measurement approach, the key is starting with building and activating against an audience segmented by zip code. Advertisers can meticulously define their target audience by leveraging a blend of client first-party and second-party data — including geo data, sociodemographic data, census data, spending habits, brand-level sales data and lifestyle preferences.
Using these geo-aware audiences enables sophisticated machine learning technology to automatically generate statistically balanced targeted and control (A/B) audience groups based on zip codes.
The algorithm scores each zip code from the target audience to find pairs of zip codes that are statistical twins — one designated for the control group and its counterpart for the targeted group. The algorithm is trained across a vast wealth of data, including demographics, household characteristics and income, enabling it to prioritize statistical balance across A/B areas.
Additionally, on-the-ground movement data is factored in to understand how people move between places and create buffer zones excluded from activation and measurement. These buffer zones help minimize the risk of cross-pollination (overlap in people moving between targeted and control areas), ensuring the A/B audiences, while being statistically similar, are made up of distinct people.
“We’re leveraging the data we know about the people who live in different zip codes to spin out the control group,” Fox said. “We find isolated clusters of zip codes spread throughout the full audience that are balanced mathematically in terms of their makeup based on a given factor, such as income. We want to ensure that our control group’s average income is similar to our exposed group’s, so we’re not predisposing A to perform better than B. We use our cross-pollination technology to eliminate audience overlay, ensuring our A/B splits are neutral and distinct.”
Lift analysis can be run across the A/B groups to measure the impact of omnichannel campaigns across various outcome indicators, such as brand lift, store visit lift, app usage lift or sales lift.
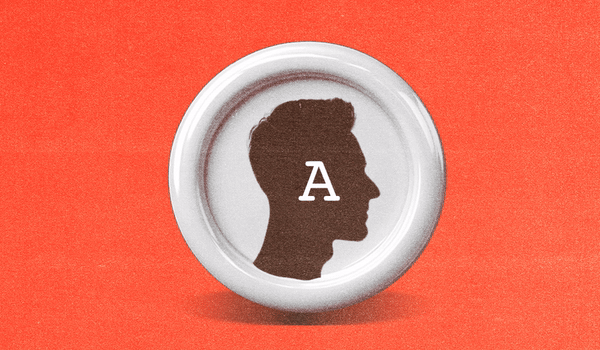
While designated market area (DMA) and regional segmentation aren’t new, this geo-based approach’s execution is novel when granularity, comparison data sets and seamless audience links are considered.
By understanding audiences at the zip code level, advertisers gain more precision than previously relied upon DMAs. Using zip codes to identify personas and determine what makes an audience unique intrinsically links this measurement method to the audience itself, making it much easier to conduct measurements and produce results.
Another important distinction is that traditional geo A/B splits utilize an entire state as a holdout rather than these carefully defined zip code level holdouts. This previous setup generates problems, like isolating a huge swathe of a brand’s audience and running the risk that the makeup in the holdout state is very different from the targeted areas, resulting in unbalanced behaviors and skewed outcomes.
At its core, this measurement method provides a clean read on the lift driven by an advertiser’s campaign. There are opportunities to leverage a brand’s in-house metrics, like new car sales or new customer acquisition, to build bespoke uplift reports from first-party data.
“The sky is the limit in theory,” said Fox. “This framework allows us to utilize those custom data sets tied back to more tangible business outcomes that are financially motivating for the brand. This helps marketers get back to proving the real-world business results they’re trying to drive.”
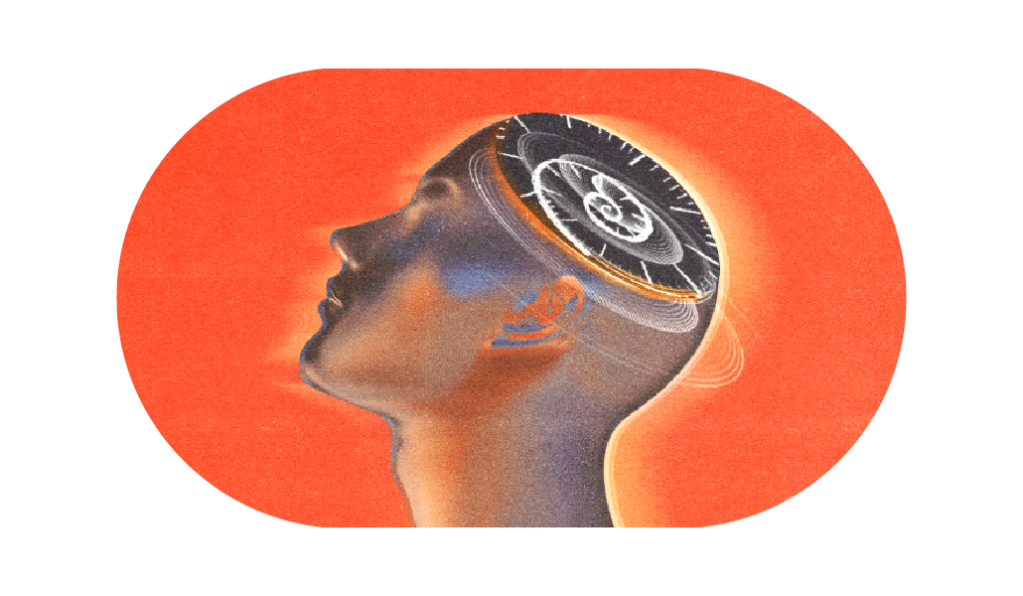
DC Lottery recently used geo-based omnichannel measurement to get a clearer picture of its audience. Working with agencies FuseIdeas and Taoti Creative, DC Lottery was eager to see campaign results, like store visits, ticket sales and brand consideration, across all channel activity. Utilizing geo-based omnichannel measurement from Blis gave the team a clearer picture of its audience as well as an understanding of campaign impact across all channels. This led to a 30% increase in overall ticket sales, a 67% increase in in-store visits and a 160% uplift in intent to purchase among customers who saw the campaign across DOOH, CTV, display, audio and online video.
“We’re interested in understanding real business outcomes that move the needle for our brand beyond just proxy metrics,” said Jayre Reaves, director of marketing and communications at DC Office of Lottery and Gaming. “Previously, there hasn’t been an offering that connects all of our campaign channels to provide holistic insight in a privacy-first way, until now.”
Confirming that a prospective provider or partner can deliver what’s expected is crucial. This is especially important when the offering, such as geo-based omnichannel measurement, is so new.
A vendor or partner’s offering needs to connect all campaign channels for holistic insights in a privacy-safe manner. Brands and advertisers should ask questions, like for a demo, to ensure the vendor can deliver.
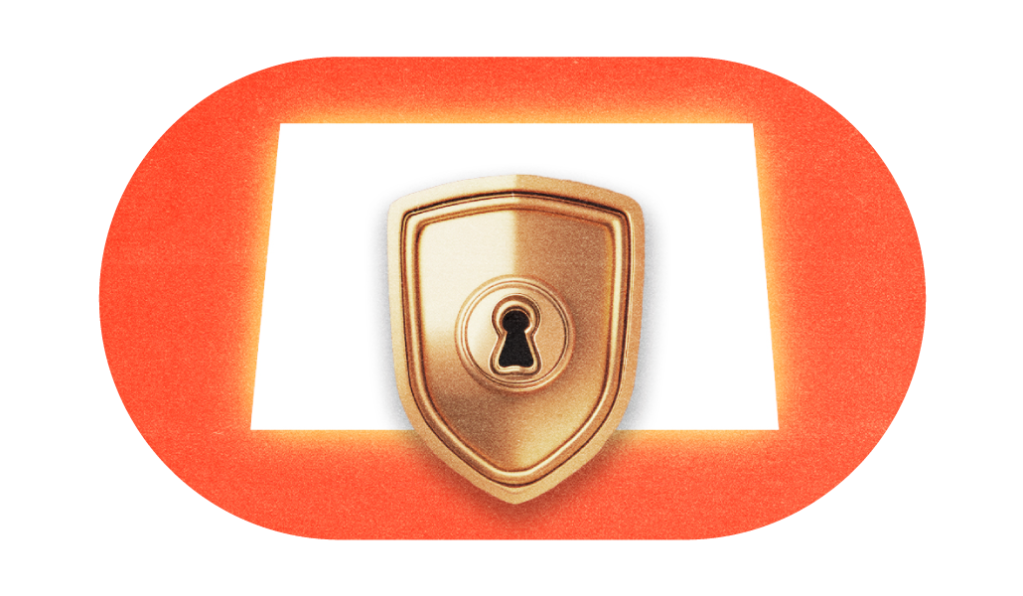
The next item on the checklist for a geo-based omnichannel measurement solution is that it’s statistically and scientifically robust. For effective campaigns using this approach, the only difference between an exposed and a control group needs to be the ad campaign itself. So, if a solution is robust enough, it should have a deep understanding of an area’s makeup and be able to create audience groups that are as similar as possible. Without that ability, any outcome differences might be attributable to the differences in each audience, rendering the campaign ineffective.
From here, the solution must be repeatable and straightforward. For example, if it’s using machine learning to create balanced, repeatable audiences, brands and advertisers should be able to easily access and execute that within a platform to enable a true test-and-learn approach. This kind of solution shouldn’t take hours and hours of data scientists crunching massive datasets that need advanced knowledge, investment and skills to complete.
After identifying suitable solutions, as brands and advertisers sort through prospective partners and providers, there are some questions they ought to ask to ensure a good fit.
Some vendors may be clinging to aspects of the industry that are technically viable but not scalable, or at least aren’t any longer. Teams should ask prospective partners how they ensure a scalable solution that isn’t based on small, niche volumes of data that won’t end up being viable to guarantee that the vendor is innovating away from such data sets.
Once brands and advertisers have chosen their provider or partner, the key to a smooth onboarding process is being as transparent as possible with each other about what outcomes they want to drive and not getting distracted by the more indirect metrics.
“The best companies will have a consultative approach,” Fox said. “Speak to the providers in the language of what you’re genuinely trying to achieve and instead of giving them a pitch that you want them to tick loads of boxes on, give them an open brief and be honest about the challenges and how they can solve those for you. That’s where you’ll see the most creativity and the best application of different solutions molding into something that’ll work for you.”
About Blis
Blis is the most scaled geo-powered advertising platform in the world, working with major agencies and their brands. Blis takes a radically different approach to omnichannel planning, buying, and measurement that’s rooted in geography, not just identity. Using AI, we understand and help show what makes audiences unique regarding where they can be found online and in the real world and how they engage with content. We can then reach and measure these audiences in a unified way across every device and channel without relying on cookies or IDs. This unique technology consistently delivers substantially better performance, scale, and buying efficiency than ID-reliant strategies alone. We’re also the only major DSP that doesn’t mark up media, meaning more of our clients’ budgets can go to work on great publishers and content. Blis was founded in the UK in 2004 and operates in over 40 markets worldwide.
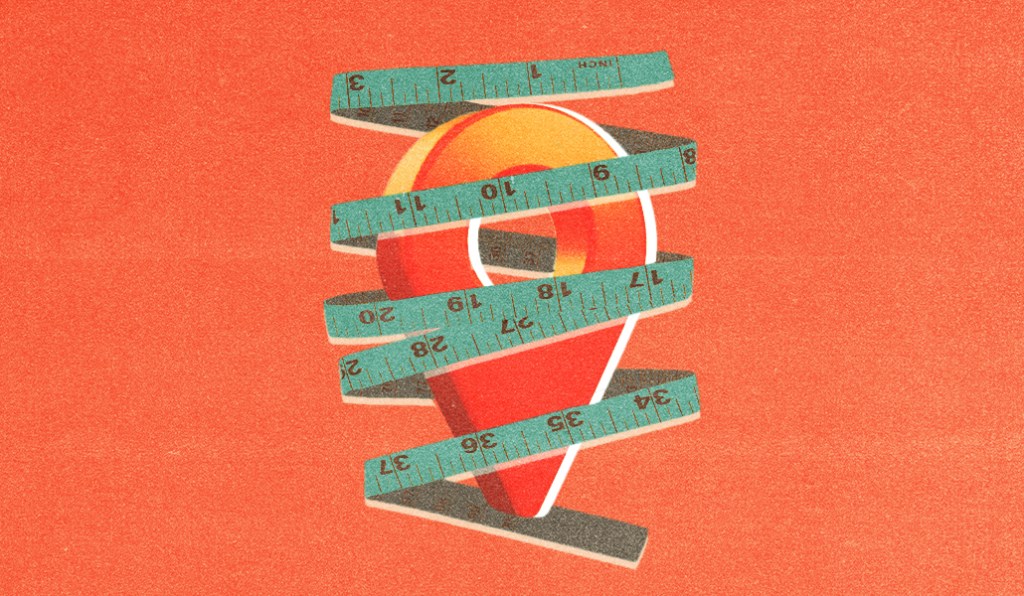