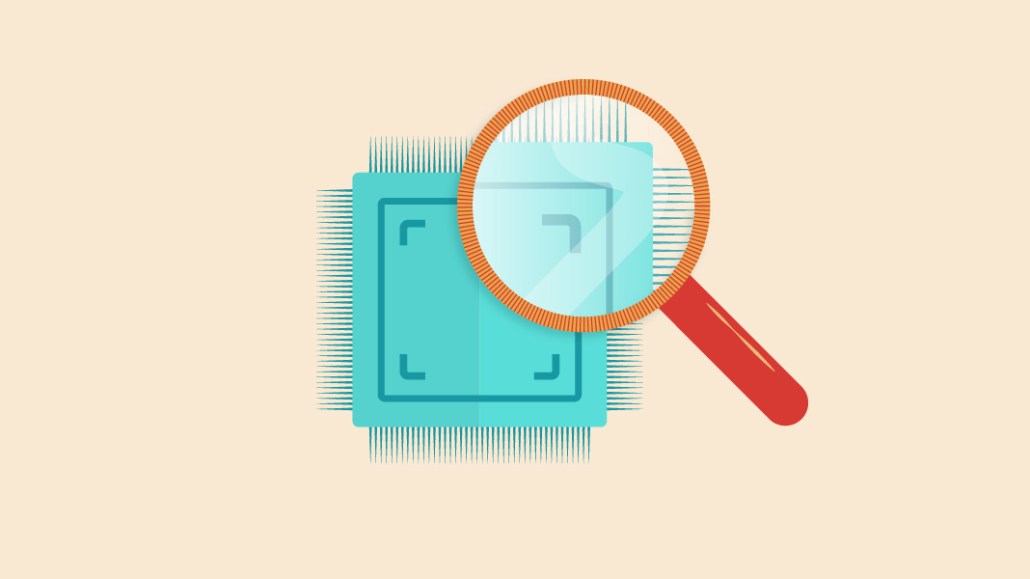
By Melinda Han Williams, VP of data science and analytics, Dstillery
In digital marketing, every brand wants to identify the best new customers at the right time on the right screen. But it’s not enough to identify a good versus a bad prospect, nor is it even enough to just understand the characteristics of a good prospect. To truly design an effective brand strategy, you’ll need to understand the variety of stories and reasons that bring customers to your brand, and then identify the different flavors of people behind each of those stories.
For example, why do people buy yogurt? The prevailing assumption is that most people buy yogurt for its health benefits. But with one leading yogurt brand, we found that consumers are drawn to yogurt for a variety of reasons, including its appeal to kids as a tasty after-school snack.
The good news is, these days brands are overflowing with data to help surface these types of unique stories. And this is true more than ever in the ad tech ecosystem’s lush landscape of observed behavioral data. AI and machine learning give us a way to use that data to surface those customer stories.
To utilize machine learning to its fullest potential, you’ll need to understand that there are two main types of the technology: supervised and unsupervised.
The difference between supervised and unsupervised machine learning
Simply put, a supervised machine learning algorithm learns what you teach it, while an unsupervised algorithm learns on its own. ‘Teaching” a machine learning algorithm means equipping it with examples of what you want it to learn so the machine is trained accordingly. Unsupervised algorithms, on the other hand, learn on their own, without any examples.
We’ve all seen each of these in action. To unlock your phone or computer with your fingerprint, you must first “teach” it what your fingerprint looks like by giving it several examples. This is supervised learning. Whereas unsupervised learning is when your photo software identifies and groups five different people that often spring up in your photos without any input from you. Both types of algorithms are incredibly powerful when wielded effectively, but each also has its limitations.
Use supervised machine learning for clearly defined questions
Supervised machine learning is a powerful tool for answering a clearly defined question when examples of the answers are readily available. For marketers, this is the most efficient way to identify new prospective customers. For example, we worked with a home décor retailer who wanted to find high lifetime value customers. We were able to use the retailer’s data on past high value customers to teach a supervised machine learning model to identify future high value customers.
Act-alike models usually employ this methodology — they are applied to build larger audiences from smaller audience segments for identifying new customers. It starts with a seed set of users who are customers of a brand, and then uses that information to build a model for predicting who will be a future customer. This approach is the best way to answer the question, “Will this individual be a customer?”
When you don’t know what to ask, use unsupervised machine learning
But what if your question is less well-defined? What if you seek to find deeper, undiscovered stories within your customer population? Supervised learning can’t help you because there are no predefined examples, and this is where unsupervised machine learning shines. Unsupervised learning allows you to answer the questions you didn’t even know to ask. By assessing a user’s entire observed behavioral data and detecting which users are most similar, unsupervised learning can discover and group these similar users — the same way your phone can group similar images of faces.
The strength of this approach is that it doesn’t rely on any preconceived ideas or examples of “who is my customer” or what different types of customers we want to see. As a result, unsupervised learning can be used to validate or disprove expectations. It can also find subpopulations or micro-audiences of users we didn’t know existed and answer the questions you didn’t know to ask. For example, for a leading fitness brand, we unexpectedly found a substantial following among pregnant women.
Machine learning has the potential to significantly augment brands’ understanding of consumers and the reasons why they buy. By understanding supervised and unsupervised machine learning, and knowing when best to employ these different approaches, marketers put themselves in a better position to reach and engage audiences.
More from Digiday
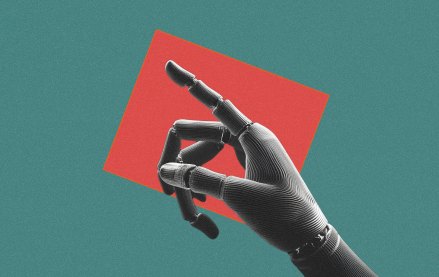
Here are the biggest misconceptions about AI content scraping
An increase in bots scraping content from publishers’ sites represents a huge threat to their businesses. But scraping for AI training and scraping for real-time outputs present different challenges and opportunities.
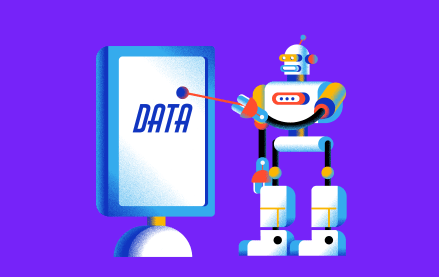
How Future is using its own AI engine to turn deeper engagement into ad dollars
Future is betting on AI to boost recirculation – and make that stickier audience more appealing to advertisers.
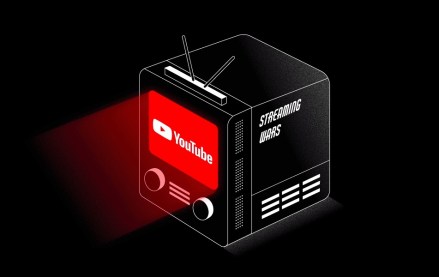
Future of TV Briefing: TV is YouTube’s top screen — except when counting views and among Gen Z viewers
This week’s Future of TV Briefing looks at how mobile still accounts for an overwhelming majority of YouTube video views and why some Gen Z viewers aren’t tuning into YouTube on TV screens.