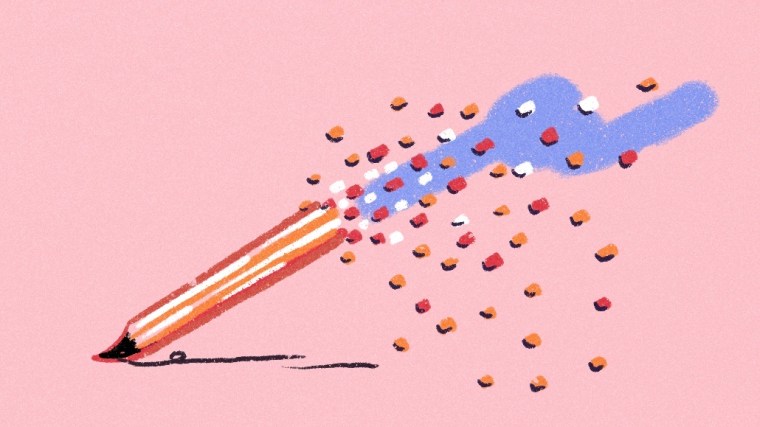
- 01 Introduction
- 02 Methodology
- 03 As AI’s text generation improves, marketers take calculated risks to adopt the tech
- 04 AI is democratizing data analysis across the board
- 05 As models proliferate and evolve, AI competition benefits marketers
- 06 A brief guide to current large language model options
- 07 Looming regulations create uncertainty for AI’s future
This research is based on unique data collected from our proprietary audience of publisher, agency, brand and tech insiders. It’s available to Digiday+ members. More from the series →
Last November, Digiday+ Research released a report from our emerging technologies series that dove into artificial intelligence applications for marketers. Shortly after it was published, the discussion around AI shifted dramatically with the release of OpenAI’s ChatGPT, which has caused a paradigm shift toward generative AI. Public access to ChatGPT allowed individuals who did not have a background in advanced computer technology to experiment with and leverage AI technology while giving them a firsthand understanding of generative AI’s potential.
“With the release of ChatGPT, we brought AI into the collective consciousness,” Mira Murati, chief technology officer at OpenAI, said in an interview with the Wall Street Journal. “And people are paying attention because they’re not reading about it in the press. People are not just telling them about it, but they can play with it. They can interact with it and get a sense for the capabilities.”
As AI becomes more mainstream with consumers, marketers have started to look to the technology as an important part of their toolkits. When comparing Digiday+ Research’s survey results from 2022 vs. 2023, marketers’ adoption rate of AI and Natural Language Processing (NLP), a branch of AI that specifically focuses on machine learning and human linguistics, has increased by 12 percentage points. To note, our 2022 data focuses primarily on NLP due it being the most widely understood form of AI at the time of our survey.
Other industry sources have reported similar increases. According to market intelligence firm International Data Corporation, AI investments are predicted to rise from $16 billion in 2023 to $143 billion in 2027. Similarly, in a Twilio survey of 2,450 business leaders, 54% of respondents said they plan to spend more on “AI-powered campaigns” next year, while 38% said they plan to use chatbots in their marketing efforts, though 28% of business leaders expressed concerns about data privacy. And a survey by Deloitte Digital found that 26% of marketers are already using generative AI, while another 45% plan to use it by the end of 2024.
As generative AI’s adoption rates have increased, marketers and brands have largely used the technology to improve their productivity. Stephen Blum, chief technology officer at API platform PubNub, said AI users are able to accomplish the same tasks they normally would, but at faster rates. “The current AI tools are exactly that — they’re productivity enhancement systems that we can take advantage of,” Blum said. “And they are a much bigger leap, like a vacuum cleaner was. Imagine what you had to do before. How did you clean your rugs before? You take them outside, wash, scrub, dry and that would take hours. But with a vacuum cleaner, you just zoom over it for a bit. And so we’re at that level with AI right now.”
In light of all of the changes to AI within just this past year, Digiday has revisited the themes covered in our previous AI report to reflect current market trends and the state of an industry in flux.
Digiday+ Research sent out a survey asking 118 respondents about current and upcoming investments in and usages of AI to map out marketers’ current applications of the technology. Digiday+ Research also conducted individual interviews with executives in the AI industry.
Year over year, chatbots and AI assistants remain the most common application of AI technology, with the majority of marketer respondents — 51% in 2023 and 78% in 2022 — selecting chatbots and AI assistants as the top NLP or AI technology their company uses.
One reason chatbots have remained at the top of marketers’ AI usage preferences is that many chatbots and AI assistants have been upgraded with higher functioning tools and capabilities as generative AI improves. For example, recent generations of chatbots are outfitted with enhanced language models that can produce more adaptive humanlike responses.
In particular, OpenAI’s ChatGPT, which debuted in November 2022, is one of the more popular versions of a regularly updated chatbot. In September, ChatGPT added new ways to “see,” “hear” and “speak” — thanks to new multimodal capabilities — and, days later, announced the platform could finally browse the web. The following month, text-to-image generator DALL-E 3 was added into ChatGPT Plus and ChatGPT Enterprise, giving users a new way to make images directly within the same platform.
Companies are taking note of the improvements and incorporating ChatGPT into their own tech. Beauty review app Supergreat, for example, has integrated ChatGPT to create its GRWM.ai chatbot on desktop that provides consumers with beauty product recommendations and videos.
Other companies have their own chatbot-enabling technology, which they’re likewise updating.
Google recently announced the release of Gemini, an update to its PaLM 2 LLM. The new large language model will power its chatbot, Bard. Similar to ChatGPT, companies can integrate Bard into their own chatbots. While Bard does have a focus on chatbots, the API associated with it also allows companies to connect it to Google Suite products, such as email, to generate summaries or other text results.
By connecting their existing AI technology or interface through ChatGPT and other LLMs’ APIs, marketers can increase their own chatbots’ capabilities to better serve and engage with customers.
Marketers’ second-most used application of AI technology is copy generation — an application also rooted in generative AI — with 43% of respondents saying they use AI for these types of tasks. And copy generation has a range of applications, everything from writing content for websites, product listings and emails to composing internal KPI reports. Streetwear brand Hat Club, for example, uses generative AI to create marketing copy for its campaigns to accelerate the content creation process.
“Our biggest challenge has always been getting our marketing copy completed in a timely manner,” said Jason Edwards, Hat Club’s e-commerce director. “The main surprise is just how close to final Attentive AI allows us to get our copy before we step in and finalize.”
Among marketers who use AI for copy generation, nearly three-quarters (71%) said they use copy generation for editorial and consumer-facing purposes. However, using AI for those purposes is not without some risk. Many marketers and industry watchdogs are concerned about copyright issues that can arise with public-facing generative AI outputs, especially when there’s a lack of transparency around what data is used to train the models.
OpenAI has been hit by a number of lawsuits regarding copyright infringement, including a class action case filed in July in which lawyers allege OpenAI violated state and federal copyright and privacy laws when collecting data used to train the language models powering ChatGPT and other generative AI applications.
Regardless of the risk, however, many companies are offering public-facing generative AI tools — like Adobe’s and Shutterstock’s image editing and generation tools — and marketers are using them. Notably, both companies offer indemnity to enterprise clients, meaning they will cover the clients’ costs if they are met with copyright-related claims or lawsuits as a result of using the companies’ generative AI tools.
Currently, most marketers are using AI copy generation for lower-risk applications like writing emails or product copy. However, some brands have moved directly into higher-profile usage. In March, Coca-Cola asked customers to use its Create Real Magic platform to generate AI images that would appear prominently on its billboards. The brand has continued the campaign into the holiday season by asking customers to create AI-generated greeting cards.
While marketers’ current top reason for using AI copy generation is to produce public-facing content, a decent proportion of respondents who said they use AI-generated copy also use it for B2B sales communication (used by 46% of respondents) or internal uses (used by 32%). As AI becomes a more capable data analytics tool, these peripheral applications of the technology are likely to see increased use.
Following copy generation, social media listening is the third-most common application of AI according to Digiday’s survey, with 38% of respondents saying their company uses AI for social media listening. Marketers turn to this application of AI because it gives them the ability to analyze information at a faster rate than many existing data analytics tools — and human analysts.
When AI is used for social media listening, the technology is trained on social data, such as likes, comments and post types, to predict what content will engage well with customers and, more importantly, to highlight emerging consumer trends. AI’s ability to highlight these trends more quickly than more conventional methods allows companies to react faster to customer needs and to ride — or attempt to avoid — the wave of consumer sentiment before it starts to decline or shift.
L’Oreal’s chief digital and marketing officer Han Wen said L’Oreal is doing more social listening this year to follow beauty trends as they emerge. The personal care company is also turning to in-house teams to accelerate content production by tapping into trends within days as opposed to older timelines that could take up to 3 months to identify and react to a trend.
“We’re recognizing the speed of change has changed,” Wen said. “It’s accelerating. Our goal within L’Oreal is really focusing on how to move at the speed of culture driven by algorithms, driven by content platforms, driven by changes around consumption behavior. In order for us to do that, it has to first start from a position where we’re listening and two, have that capability in-house for us to activate very, very quickly.”
Overall, one of the biggest changes in the last few months when it comes to AI has been the paradigm shift from marketers building AI tools to marketers training third-party algorithms on in-house data. Given that an AI algorithm that analyzes social conversations can be difficult to develop from scratch, most marketers opt for the many open-source or licensed options now available. Ultimately, many AI practitioners say the data used to train the models is more important than which model is selected.
“Usually, when you’re talking about AI, the biggest moat that you can have is not the algorithm, because all that is open source,” said Stephen Blum, chief technology officer at API platform PubNub. “Even Google says ‘take it all, you can have it all.’ The algorithm is free, but the data is where the IP is.”
AI can also be used to highlight trends beyond those identified through social media listening. Models can be trained using sales, ad and customer review data, among other sources. And generative AI has opened up a lot of possibilities for marketers due to its ability to sift through and analyze a vast amount of data in a short period of time.
One key innovation is that many of the generative AI tools currently available provide a conversational interface, akin to chatbots. Instead of using tools built for manual data manipulation, AI models trained on data are able to generate analysis in the form of text responses to specific prompts. This interface replaces hard skill sets like data formatting or query languages and provides a flexibility that did not previously exist. Users only need to understand what result they want and to be able to craft a natural language prompt to elicit that result — giving marketers a lower barrier of entry to AI-based data analysis.
Mary Grygleski, senior developer advocate at real-time data company for building production generative AI applications DataStax, said one challenge within the existing AI scenario, however, is having a data storage solution that can scale. She said most companies are using private cloud storage systems instead of in-house databases. Private cloud storage can be more cost-efficient, but it doesn’t allow for as much control over infrastructure as in-house databases do. As data becomes more important for AI tools, companies have to think more carefully about how to store data and if their infrastructures can keep up with AI systems and properly power their functions.
“As an analogy, we all go out and buy houses and we look at beautiful houses, but may not pay attention to all the things that are underneath — the wiring of these things,” Grygleski said. “The behind-the-scenes are very important. ‘Can I have all the lights on in my house?’ ‘Can it pass all of the system stress-testing?’”
“Likewise, an AI system deals with so much data,” she added. “Large language models store a humongous amount of data, but despite its size, the data isn’t sufficient because it doesn’t take into account your most updated domain-specific data. Those things all need to work together. … The data is one big challenge for any AI system.”
Since 2022, the percentage of marketers working with third-party vendors for AI solutions has increased from 53% to 62%, Digiday’s survey found. And the proportion of marketers both building in-house tools while also using third-party vendors has decreased from 31% to 20%. Many marketers have opted to outsource their tool-building needs rather than build the tools themselves, as third-party AI solutions have become incredibly polished and sophisticated over the last year.
Savio Rodrigues, vp of ecosystem engineering and developer advocacy at IBM, said he’s noticed a greater focus recently on skilling and offering embeddable AI technology. “There is a tech skills shortage, and most businesses also do not have the time and financial resources to build, manage and provide the ongoing support required to develop these AI models from scratch,” Rodrigues said.
In fact, there is an ongoing race among tech companies to create the latest and greatest AI tools for a growing market of clients. Recently Anthropic, an AI startup founded by former OpenAI employees, launched a new version of its chatbot, Claude, intended to rival ChatGPT. The upgrade allows Claude to process more information by adding more API tools, which increases its ability to give accurate answers.
Last month, Inflection AI, another AI startup, released a new language model that it claims outperforms other LLMs such as Google’s PaLM. Also in November, Microsoft, which has hired many former OpenAI employees, released Orca 2, a smaller language model. Meanwhile, Meta has its LLAMA-2 language model.
While marketers are benefiting from tech companies’ competition to build better AI models, they may face decision paralysis from the constant updates and new options and upgrades the companies make available. However, as AI options continue to grow, marketers are likely to become more accustomed to regularly diversifying their AI tool kits with niche solutions.
DataStax’s Grygleski said she believes customers are benefiting from the tech race to create the latest AI tools as well. “It gives consumers more choices,” she said. “We’re seeing more integrations between different vendors and, instead of [them] just saying ‘mine is better,’ they’re finding ways to bring out the best of all worlds.”
One item to note from Digiday’s survey results is the 18% of marketer respondents who said that they build AI tools in-house. Outside of some larger enterprise companies and AI firms, most companies typically do not have a dedicated AI team. For those respondents who said they are building AI tools in-house, most of them are likely using an existing open-source tool like OpenAI and training it on their own data — not to be confused with building a home-brewed AI model.
Given the proliferation of AI options, this section of our report provides brief overviews of some of the current Large Language Models (LLMs) that help power AI tools with the aim of identifying key biases or emerging strengths as marketers make their vendor decisions. For more information, and definitions of AI terms, please refer to Digiday’s AI Glossary.
Adobe
- Name: Adobe Sensei
- Access: Not open source and the model is not available for public use, but it powers tools that are available for commercial usage.
- What makes it stand out: Adobe Sensei powers many Adobe Suite products such as Analytics and Firefly, Adobe’s image-based generative AI tool.
- Notable tools: Firefly is Adobe’s main tool. It can be integrated into Adobe Photoshop and Illustrator. It not only allows for image generation, but also image editing.
AssemblyAI
- Name: LeMUR
- Access: Open source and does have a pay-for-what-you-use pricing structure.
- What makes it stand out: It is a standout in voice recognition, but unlike Amazon’s Alexa LLM has more flexibility and it is not tied to the Amazon product suite. However, it does not have access to the same amount of voice data as Amazon’s Alexa.
- Notable tools: LeMUR powers two tools: speech-to-text transcription and Audio Intelligence. Speech-to-text transcription is a transcription tool that also offers customizations such as removing filler words and profanity filters. Audio Intelligence is an analysis tool that provides speech sentiment analysis and can also provide summarizations of spoken content.
Amazon
- Name: Alexa LLM
- Access: Not currently available for public use.
- What makes it stand out: It is a standout in voice recognition and will also allow Alexa to connect with other APIs to perform customized functions.
- Notable tools: Alexa LLM will be mainly integrated with its namesake device, the Alexa assistant. The integration will allow Alexa to perform more functions and also to develop personalities when engaging with users. Amazon is one of the main companies to integrate AI into real-world devices — with another notable example being Google.
Anthropic
- Name: Claude 2
- Access: Open source and does have a pay-for-what-you-use pricing structure.
- What makes it stand out: Anthropic’s ethos is to build safety-oriented AI models, meaning Claude 2 focuses more on brand safety and will likely emphasize adherence to future regulations.
- Notable tools: Anthropic also provides an API that allows businesses to connect to Claude 2 to power other chatbots. Along with chatbots, the API is able to power text generation and auto-code writers.
- Name: PaLM 2
- Access: Open source and free for both research and commercial use.
- What makes it stand out: Along with access to Google’s expansive, ever-growing dataset, this LLM has specialties in multilingualism, reasoning (which includes logic and mathematics) and coding.
- Name: Gemini
- Access: At the time of writing, Gemini was newly announced and not yet open source or available for commercial use.
- What makes it stand out: It has the ability to take in text, images, video, audio and code as prompts, allowing for more flexibility.
- Notable tools: Vertex AI is a platform available on Google’s Cloud service that allows users to train and launch machine learning and AI tools. The tool also allows for monitoring post-launch to track its performance. Currently it is powered by PaLM 2, but will later also include Gemini.
Meta
- Name: Llama 2
- Access: Open source and free for both research and commercial use.
- What makes it stand out: This LLM is trained on Meta’s incredibly large dataset as well as publicly available information. According to Meta’s website, “Llama 2 is not trained on Meta user data.”
- Notable tools: PyTorch is an open-source framework that allows others to create, train and test their machine-learning tools. The tool is also connected to Meta’s datasets. As with Llama 2, PyTorch is also free and available for research and commercial use.
Microsoft
- Note: Microsoft’s tool is not itself an LLM, but works to improve them.
- Name: Orca 2
- Access: Open-source and free for research only.
- What makes it stand out: It is a tool designed to improve smaller LLMs and specializes in creating synthetic data to train smaller language models. Essentially, this tool creates a database that smaller language models can use to create more responses.
OpenAI
- Name: GPT4
- Access: Not open source and does have a pay-for-what-you-use pricing structure.
- What makes it stand out: It is one of the most commonly recognized LLMs and has become somewhat synonymous with generative AI. It specializes in text generation. It also powers DALL-E 3, which focuses on image generation and is also a part of ChatGPT.
- Notable tools: OpenAI API allows users to build other chatbots, AI assistants and other applications by leveraging GPT4’s LLM. The API has been used to create other tools as well, such as sentiment analyzers, computer code debuggers and auto-code writers.
While AI adoption is accelerating rapidly, regulations and policies will undoubtedly play a critical role in how the technology is implemented in the future. Currently, most legislative bodies are in a learning phase in which they are trying to understand the technology and its potential threats. For example, the U.S. Copyright Office is conducting a study on copyright issues that have arisen from generative AI being trained on licensed and copyrighted information and images. Similarly, U.S. regulators are exploring how generative AI could impact various industries and consumers more broadly. On Sept. 22, the Federal Trade Commission hosted a virtual roundtable to address copyright concerns and other issues with various authors, artists and other participants.
While some laws that cover AI have been put into place, most of them are generalized and don’t touch on AI’s specific capabilities or the content it can produce. Both the Center for AI and Digital Policy (CAIDP) and The White House have created frameworks for the general development of AI technology. The CAIDP has endorsed the U.S. AI Act, which aims to create a high-level framework for U.S. regulations for AI. And the White House has issued an executive order on the development and use of AI technologies that emphasizes the need for such technologies to be safe and secure — though the order does not go into further detail about what would constitute safety or security. Additionally, current laws have not kept up with AI’s rapid growth and, as a result, lack proper rigor.
DataStax’s Grygleski pointed to the need for a regulatory body to oversee data access. “This is definitely an area that still needs a lot of work, especially now that we’re dealing with these publicly owned companies that have access to all your data,” Grygleski said. “There definitely needs to be a watchdog organization and monitoring agencies, a regulatory body that governs the data access and storage. … I’m seeing efforts towards that, but it’s not quite mature.”
Regulations could very well be the main barrier going forward for marketers seeking to adopt AI technology. With uncertainty surrounding what AI tactics may be allowed in the future and general brand safety concerns, some marketers have as of yet been unwilling to make too great of an initial investment in the technology and, therefore, put themselves at the forefront of AI adoption. Instead, for now, many may opt to invest in AI applications that have proven track records and straightforward applications without delving into the murkier privacy ethics.